Library:THE PEOPLE’S REPUBLIC OF WALMART: Difference between revisions
More languages
More actions
RedCustodian (talk | contribs) No edit summary Tag: Visual edit |
RedCustodian (talk | contribs) No edit summary Tag: Visual edit |
||
Line 241: | Line 241: | ||
== MAPPING THE AMAZON == | == MAPPING THE AMAZON == | ||
Amazon is on its way to developing psychic powers. Or at least, such was the fantasy that one could be forgiven for believing, based on the hosanna-filled, adrenalized newspaper column inches that appeared in the summer of 2014 when the online bookseller-turned-“everything store” filed a patent application for a new process it called “anticipatory shipping.” Amazon would soon know what you wanted to buy before you knew it yourself. When you placed an order for the latest John Green young adult novel for non–young adults, another jar of artisanally brined lupini beans, or that Instant Pot wonder–pressure cooker that produces pulled pork faster than the speed of light, the package would already be on its way. | |||
As those journalists less prone to the confection of hyperbolic clickbait pointed out at the time, what this patent describes is in truth a very small step from what Amazon already does. It is a minor extension of the kind of data the company already collects and of the colossal, tentacular logistics operation it already runs. Amazon, building its retail market position on the back of the internet revolution, is the largest technology company using the fruits of modern IT to distribute consumer goods. In short, Amazon is a master planner. It is these sorts of logistical and algorithmic innovations that give the lie to the hoary free market argument that even if planning can deliver the big stuff like steel foundries and railways and healthcare, it would stumble at the first hurdle of planning for consumer items. A fortiori, Amazon offers techniques of production and distribution that are just waiting to be seized and repurposed. | |||
=== What Amazon Plans === | |||
Since its late-’90s dot-com beginnings selling only books, Amazon has expanded to potentially fulfill a large part of a household’s everyday consumption. Echoing Walmart’s horizontal integration, the company has even started to incorporate producers of the things it sells into its distribution network by placing its own workers at the factories and warehouses of some of its key suppliers. Under what the company calls its “Vendor Flex” program, the number of Band-Aids that Johnson & Johnson produces, for example, can depend in part on Amazon’s need. It gives the retail behemoth a role in managing production that extends beyond its own corporate borders. | |||
Beyond simply distributing products, Amazon is, like Walmart, “pulling” demand. In fact, in its early days, Amazon headhunted so many top-level managers from Walmart for their logistics savvy that the Waltons sued. The untold billions of gigabytes of customer data that Amazon collects and the algorithm marvels it uses to parse this data give it an incredibly detailed picture of what people want to buy, and when. Meanwhile, integrating operations with producers ensures that products can be ready in sufficient quantities. Here too, given the sheer scale of this economy, we see the fits and starts of a more integrated model of production and distribution planning, however hierarchical and servile toward its bosses it may be. We might describe Jeff Bezos as the bald, moustache-less Stalin of online retail (editor note: no we may not!). | |||
Yet at heart, Amazon remains (for now) a giant distribution network for consumer goods. The internet age has enabled the rise of a new type of retail model for moving goods from producers to consumers, and Amazon took advantage of this opening better than any of its rivals did. Amazon now controls nearly half of total online retail in the United States. So when Amazon plans, it plans big. Some of Amazon’s planning problems are the same as those faced by other major distribution networks; other problems are entirely novel. In essence though, Amazon’s story is another tale of getting the logistics right—in other words, getting things from point A to point B as cheaply as possible. While this task sounds simple enough, it demands plans for everything from warehouse siting and product organization to minimizing the costs of delivering customers’ packages and shortening delivery routes. Wired magazine describes the company as “a vast, networked, intelligent engine for sating consumer desire.” | |||
Add to this the fact that Amazon, as with every internet company, collects improbable amounts of data on its consumers. A conventional brick-and-mortar store doesn’t know which products you look at, how long you spend looking at them, which ones you put in your cart and then put back on the shelf before arriving at the checkout, or even which ones you “wish” you had. But Amazon does. This data tsunami not only involves consumer information, but stretches throughout the supply chain, and the company uses this data to its advantage wherever it can. Its planning problems are no longer the pedestrian optimization challenges faced by any large company before the internet age, but rather the optimization of “big data”—sets of data that are produced at such gargantuan volumes, varieties and velocities that traditional data processing techniques and software are insufficient. | |||
Amazon’s scale—its ambition to be the “everything store”—introduces significant problems for its IT systems. It is one thing to deliver even a thousand products to a hundred or a thousand retail stores, as would a traditional seller. It is another to deliver millions of products to millions of customers. | |||
The warehouse and transport problems mentioned above are a particular class of mathematical challenge known as “optimization problems.” In an optimization problem, we aim to do something in the best way possible, subject to a number of limits on our action, or “constraints.” Given three different possible routes through a city to deliver a package, say, which is fastest given the number of traffic lights and one-way streets? Or more realistically for Amazon, in delivering some daily number of packages, the company is limited by the schedule of delivery flights, the speed of airplanes, the availability of delivery trucks and a host of other constraints, in addition to city traffic. There are also random events, such as bad weather, that can shut down airports—and while these are sporadic, they are also more likely in some places and at certain times than others. | |||
Every day when you commute to work, you are solving a relatively simple optimization problem. But the math behind optimization is very complex for problems with more than even just a handful of constraints. Given enough variables (conditions that can change) to be optimized and enough constraints, even the most powerful supercomputer we can currently construct, armed with the best possible algorithm we can design, would be incapable of solving some of these problems within our lifetime, and some even within the lifetime of the universe (editor's note: there’s a $1 million reward left unclaimed for anyone who can solve the century-old “traveling salesman” problem!). Many of Amazon’s problems fall squarely into such categories. | |||
So while patents for drone delivery get all the media attention, the true wonders at the heart of its operations are actually the esoteric mathematical techniques that help it manage and simplify its optimization problems. To give one example, these key patents help Amazon plan how to best move items between warehouse shelves and customer doorsteps. Part of solving this problem involves “load balancing”: the same way that your computer shifts tasks so as to not crash any single system, Amazon decides where to build its massive warehouses and how to distribute products between them to make sure no part of its system gets overloaded. To be clear, Amazon’s planning methods are not complete solutions to optimization problems that might take the lifetime of the universe to solve, but instead simply best approximations to get around exploding mathematical complexity. Yet Amazon still chooses to plan rather than leave optimization up to price signals from the market. | |||
Again, take the problem of shipping orders at the lowest cost. Even precisely answering the seemingly simple question of finding the lowest cost shipping method for a day’s worth of orders can quickly grow out of hand. There is no single best way to ship one order out of thousands or millions shipped on a given day, because each order’s cost depends on all the others. Will the plane from the UPS “Worldport” hub in Louisville, Kentucky, to Phoenix be full? Did your neighbor down the street order her electric toothbrush with express shipping, or can it be delivered with your book order tomorrow? The complexity ratchets up still further when Amazon considers not only all the possible alternative routes—which it controls—but also adjusts for the possibility of random events such as severe weather and tries to predict the next day’s orders. This “order assignment” optimization problem has hundreds of millions of variables, and no easy solution. The problem is so complex that there are not even approximations that can take every aspect of the problem into account. | |||
But despite such problems, the planning process within Amazon does not fall apart. While Amazon may depend on horrible working conditions, low taxes and poor wages, it nevertheless functions. The planning problems faced by individual corporations under capitalism do have approximate, “good enough” solutions. As this book argues again and again, planning exists on a wide scale within the black box of the corporation—even if it is “good enough” rather than perfect. | |||
That’s the trick: to find the best possible, even if partial, approximations. Amazon’s modelers work to bring intractably complex problems down to size, to build plans that neither stretch into infinite time, nor respond to all the possible random events that could happen at every step, but that simply work. | |||
=== Structure amid Chaos === | |||
Describing Amazon as a big planning machine doesn’t quite match its image as an icon of “new economy” disruption. Even before Silicon Valley became a hub of global capitalism, planning was typically well hidden behind the facade of competition. Today, the facade has only become more ornate: all you see is a website and then a package at your doorstep. Behind the scenes, however, Amazon appears as a chaotic jumble of the most varied items zipping between warehouses, suppliers and end destinations. In truth, Amazon specializes in highly managed chaos. Two of the best examples of this are the “chaotic storage” system Amazon uses in its warehouses and the recommendations system buzzing in the background of its website, telling you which books or garden implements you might be interested in. | |||
Amazon’s recommendations system is the backbone of the company’s rapid success. This system drives those usually helpful (although sometimes comical—“Frequently bought together: baseball bat + black balaclava”) items that pop up in the “Customers who bought this also bought…” section of the website. Recommendations systems solve some of the information problems that have historically been associated with planning. This is a crucial innovation for dreamers of planned economies that also manage to satisfy consumer wants. The chaos of individual tastes and opinions is condensed into something useable. A universe of the most disparate ratings and reviews— always partial and often contradictory—can, if parsed right, provide very useful and lucrative information. | |||
Amazon also uses a system it calls “item-to-item collaborative filtering.” The company made a breakthrough when it devised its recommendations algorithm by managing to avoid common pitfalls plaguing other early recommendation engines. Amazon’s system doesn’t look for similarities between people; not only do such systems slow down significantly once millions are profiled, but they report significant overlaps among people whose tastes are actually very different (e.g., hipsters and boomers who buy the same bestsellers). Nor does Amazon group people into “segments”—something that often ends up oversimplifying recommendations by ignoring the complexity of individual tastes. Finally, Amazon’s recommendations are not based on simple similarities, such as, in the case of books, keywords, authors or genres. | |||
Instead, Amazon’s recommendation algorithm finds links between items based on the activity of people. For example, a bicycle repair manual may consistently be bought alongside a particular bike-friendly set of Allen keys, even though the set isn’t marketed as such. The two things may not be very obviously related, but it is enough that some people buy or browse them together. Combining millions of such interactions between people and things, Amazon’s algorithm creates a virtual map of its catalog that adapts very well to new information, even saving precious computing power when compared to the alternatives—clunkier recommendation systems that try to match similar users or find abstract similarities. | |||
Here is how the researchers at IBM’s labs describe Amazon’s recommendations: “When it takes other users’ behavior into account, collaborative filtering uses group knowledge to form a recommendation based on like users.” Filtering is an example of an IT-based rejoinder to one of the criticisms Hayek leveled against his socialist adversaries in the 1930s calculation debate: that only markets can aggregate and put to use the information dispersed throughout society. The era of big data is proving Hayek wrong. Today’s deliberately planned IT systems are starting to create “group knowledge” (collective intelligence, or shared information that only emerges out of the interactions within or between groups of people) out of our individual needs and desires. And Amazon doesn’t just track market transactions. Beyond what you buy, the company collects data on what you browse, the paths you take between items, how long you stay on the page of each item you browse, what you place in your cart only to remove it later, and more. | |||
Hayek could not have envisioned the vast amounts of data that can today be stored and manipulated outside of market interactions (and, to be fair, even many Marxists have assumed that the myriad capricious variables associated with faddish consumer items in particular forecloses the capacity for their socialization), although he certainly would have admired the capitalists such as Bezos who own the data and use it to pad their obscene fortunes. It is a delicious irony that big data, the producer and discoverer of so much new knowledge, could one day facilitate what Hayek thought only markets are capable of. | |||
Really, it is not such a big step from a good recommendation system to Amazon’s patent for “anticipatory shipping.” It has a viability beyond any Silicon Valley, TED Talk–style huckster bombast or tech-press cheerleading. The reason this genuinely incredible, seemingly psychic distribution phenomenon could actually work is not a result of any psychological trickery, subliminal advertising craftiness, or mentalist power of suggestion, but is found in something much more mundane: demand estimation. With its huge data sets that measure the relationships between products and people, Amazon is already very successful in figuring out demand for particular products, down to a previously unimagined level of detail. | |||
In our irrational system, the ultimate purpose of product recommendations is to drive sales and profits for Amazon. Data scientists have found that rather than high numbers of customer-submitted reviews, which have little impact, it is recommendations that boost Amazon’s sales. Recommendations help sell not only less popular niche items—when it’s hard to dig up information, even just a recommendation can be enough to sway us—and bestsellers that constantly pop up when we’re browsing. | |||
Zooming out beyond Amazon’s corporate interests, the recommendations system is a way of managing and integrating great swaths of social labor. Many of us freely, without expectation of any reward, spend time and energy writing reviews and giving out stars to products or even just mindlessly browsing on Amazon and other technology platforms. This is work that we and others benefit from. Even over the course of one day, we may repeatedly engage in unpaid labor to rate everything from the relatively innocuous, such as call quality on Skype, to the more serious, such as posts, comments and links on Facebook and Twitter, to the potentially very impactful on individual lives, such as the “quality” of Uber drivers. Under capitalism, the social labor of many is transformed into profit for the few: the filtering may be “collaborative,” but the interests it serves are competitive and very private. | |||
=== Workers Lost in the Amazon === | |||
While many of us end up using free time to perform the social labor that allows Amazon to perfect its recommendations system, Amazon’s warehouses run on paid labor that is nonunionized and frequently occurs under appalling, similarly big data–disciplined conditions. Before taking a closer view of the work itself, let’s quickly look at the workplace. The focal points of Amazon’s distribution network are its warehouses, which the company calls “fulfillment centers.” These usually take up football fields’ worth of floor space jammed with shelving units. Amazon uses a peculiar form of organization called “chaotic storage,” in which goods are not actually organized: there is no section for books or subsection for mystery fiction. Everything is jumbled together. You can find a children’s book sharing a bin or shelf with a sex toy, caviar next to dog kibble. | |||
Once again, powerful planning is what allows Amazon to save on what turns out to be needless warehouse organization. Every item that enters a fulfillment center gets a unique barcode. Once inside the warehouse, items go in bins, each of which also has a unique code. Amazon’s software tracks both the items and the bins as they move through the warehouse. The software always knows which bin an item is in and where that bin is. Because items can always be found easily, deliveries from suppliers can be unloaded where it is convenient, rather than methodically organized and reorganized. | |||
Amazon’s chaotic storage could be a metaphor for the free market system: at first glance, it seems that the chaos organizes itself. Orders and packages zoom through the system and customers get what they want. But as with the free market, upon closer inspection we see thickets of deliberative planning at every step. Highly refined IT systems make sense of the chaotic storage, track items from the moment they arrive at a warehouse to the moment they leave, and make sure everything falls seemingly supernaturally into place. Everything ordered, coordinated, planned and not a market in sight to perform any of these billions of allocation decisions. | |||
Planning is also present in the most minute details of a warehouse worker’s day. Handheld scanning devices tell workers where to go to pick items for orders. Workers are appendages of machines that lay out precisely which routes to follow between shelves and how long they should take. Here’s how a BBC undercover worker-reporter described the work: “We are machines, we are robots, we plug our scanner in, we’re holding it, but we might as well be plugging it into ourselves.” A leading UK researcher on workplace stress contacted by the same BBC investigation claimed that conditions at Amazon warehouses pose serious physical and mental health risks. | |||
Around the start of this decade, Amazon’s top operations managers determined that its warehouses were still too inefficient, and so they themselves went shopping for something better. In 2012 Amazon bought Kiva Systems, a robotics firm, and it now uses robots to put its entire shelving system into motion. Amazon’s updated, even more automated fulfillment centers now feature shelves that move and humans who stand in place—the opposite of what a warehouse normally looks like. Flat, Roomba-like robots rove the warehouse floor along designated pathways. They can lift entire shelving units just off the ground and maneuver them along the same pathways to “picking stations.” These are small designated areas where human order pickers stand, taking items from storage bins and putting them into order bins as shelving units come and go. Frederick Taylor would be proud! | |||
The social, physical and mental cost of a machine for delivering the right things to the right people ultimately falls on the workers who make the machine hum—regardless of whether workers are piloted around a maze of shelves by a handheld scanner or pick orders in place while robots whiz to and fro toward them. The boosters at Wired magazine are in awe of the subjugation of the Chaplins in this twenty-first-century Modern Times: “The packing stations are a whirl of activity where algorithms test human endurance.” | |||
Other more critical reporting has been less kind to Amazon in fleshing out just what these endurance tests entail. In 2011 the Lehigh Valley, Pennsylvania, local paper, the Morning Call, investigated its nearby Amazon fulfillment center. Workers said they routinely faced impossible-to-meet targets, debilitating heat and constant threats of being fired. On the hottest days of the year, Amazon had paramedics on hand outside the warehouse to treat heat-exhausted workers—a cheap Band-Aid solution for Amazon that makes clear its low estimation of health and safety; apparently humane working conditions are not one of its algorithms’ optimization constraints. It was only after this story blew up in the national media and the revelation hurt its largely liberal-tech-and-innovation brand image that Amazon began to refurbish some warehouses with air conditioning. In fact, only one out of the twenty workers featured in the Morning Call story said Amazon was a good place to work. | |||
Amazon workers interviewed by the media consistently report feeling the constant stress of surveillance. Being too slow to pick or pack an item, or even taking a bathroom break that is too long, results in demerit points. Amassing enough of these points can lead to being fired. Soon, this feeling of constant surveillance could become far more visceral: in February of 2018, Amazon patented a wristband that, like an episode of Black Mirror, monitors a warehouse worker’s every hand movement in real time. And Amazon pits workers not only against the clock, but also against one another. Utilizing the lumpenized and semi-proletarian masses from among the industrial reserve army, Amazon staffs warehouses with a mix of permanent and temporary workers hired by subcontractors. Permanent positions are few, but they come with some security, slightly higher pay and limited benefits; they are dangled as carrots before temporary workers, encouraging competition and overwork, further fostering a climate of uncertainty and fear. | |||
With the help of robots, the average time to fill an order in a warehouse automated by Kiva technology has plummeted from ninety minutes down to fifteen. Working conditions, however, haven’t budged: the work remains as dull and draining as ever, warehouses remain hot, and the pace of work can be absurdly fast, regardless of the level of automation. While workers in automated warehouses stand all day and try to keep up with the robots zooming by, workers in the non-automated warehouses can expect to walk nearly double the distance on a daily shift of a typical mail carrier. Even small things like distances to break rooms can be an obstacle—sometimes so long that going both ways can take up most of a break. | |||
Long hours for low pay are the norm in an Amazon warehouse, but the relatively highly paid white-collar workers at Amazon also face a crushing work environment. A 2015 New York Times exposé revealed an environment of overwork and “purposeful Darwinism” that pushes many past their physical and emotional limits. Even if sophisticated planning is Amazon’s workhorse, it is implemented within the bounds of a ruling ideology of ruthless competition that breaks white-and blue-collar workers in different ways. Put differently, Amazon is doing exactly what Marx described in a passage from The Communist Manifesto that often goes underappreciated: “The bourgeoisie cannot exist without constantly revolutionizing the instruments of production, and thereby the relations of production, and with them the whole relations of society.” Our task must be to disentangle the good brought by technology from the tentacles of a social system that degrades workers and subverts more rational planning. | |||
=== Amazonian Technologies beyond Amazon === | |||
Despite being a model of the new, disruptive, internet-dependent capitalism, Amazon remains a planning device as much as other companies ever have. In simplest terms, Amazon is a giant planned machine for distributing goods. It is a mechanism for forecasting, managing and meeting demand for an incredibly wide array of things we need and want. It is a collection of thousands of interlocking optimization systems that work together to carry out the deceptively simple task of moving objects from producers to consumers. Rather than the anarchy of the market, once we enter the Amazon, we are entering a sophisticated planning device—one that offers not only clues for how we could manage demand and supply of consumer goods in a society not built on profit, but also warnings to would-be planners for the public good. | |||
Instead of optimizing the satisfaction of our needs and desires, as well as workers’ working conditions and livelihoods, Amazon’s plans are geared toward maximizing profit for its shareholders—or future profit, since Amazon keeps plowing money from sales into research, IT and physical infrastructure to squeeze out competitors. Planning for profit is in fact an example of capitalism’s web of allocation inefficiencies. The planning technologies dreamed up by Amazon’s engineers are a way of meeting a skewed set of social needs—one that ends up enriching a few, misusing substantial free social labor, and degrading workers. A democratized economy for the benefit of all will also need institutions that learn about people’s interests and desires, optimize via IT systems, and plan complex distribution networks; but they will look different, perhaps alien to the systems we have today, and they will strive toward dissimilar goals. | |||
Three challenges should give us pause before even beginning to call the riddle of democratic planning solved. | |||
First, there is large-scale technical feasibility. The difficulty of planning and optimizing even the isolated task of delivering Amazon’s packages demonstrates that designing systems for economy-wide planning will be anything but trivial. The algorithms that power everything from Amazon’s recommendation system to Google’s search engine are still in their infancy—they are relatively simplistic, making best-estimate guesses, and are prone to failure. Algorithms run into systemic problems, for example with working class and poor people who more frequently use shared devices to shop or non-English speakers, where their capacity for “reading” nuance is limited. We’ll have to storm both the barricades and the optimization problems. | |||
Second, the planning done by Amazon and others still relies heavily on prices in interactions that take place beyond the borders of the firm itself. Amazon purchases its inputs—from the multitude of items it stocks, to the warehouse shelves they sit on, to the servers that run its database—on a market; consumers, meanwhile, also take into account the relative costs of items when deciding whether to add them to their virtual carts. Beyond the confines of the firm, a market system continues to operate. This means that it’s not simply a matter of repurposing existing technologies, lopping off the bosses and otherwise keeping everything the same. Even though there is market-less planning within corporations, it is a form of hierarchical, undemocratic planning that is very much necessary to survive and thrive in a market. Many elements of this planning apparatus, their very form and purpose, are conditioned by that undemocratic hierarchy. | |||
Finally, while the big data collected and processed by Amazon is precisely the kind of tool that would aid in overcoming these challenges of large-scale economic calculation—and indeed it is already being used in this way by the Amazons and Walmarts (never mind the Facebooks and Googles) of the world—we have to recognize that alongside the staggering freedom-enhancing potential of the massive data sets held by both corporations and states, there also lies a staggering capacity for freedom restriction. | |||
The story of Walmart’s major rival Target sending deals on diapers and baby food to several expectant mothers who did not yet themselves know they were pregnant, based on data mined on individual spending patterns, seems almost quaint today. Now, we are only a single Google search for “poor sleep” away from months of bombardment by mattress ads on every social media network to which one belongs. There are more insidious examples: in 2012, the short-lived “Girls Around Me” app used a mash-up of geolocation and social media data to allow individuals to find out all kinds of personal details about women in their vicinity who had used Facebook or Foursquare’s “check-in” feature. The UK’s Daily Mail called it the “Let’s Stalk Women” app, while science fiction author Charles Stross imagined a near future of other, far-nastier data mash-ups—could neoNazis create a “Jews Near Me” app? Beyond the private sector, states across the world are also increasingly using and misusing big data. Police departments across the United States have begun to experiment with something called “predictive policing” to devise methods for predicting offenders, victims, identities, and locations of crimes. It is the arrival of “pre-crime” from the pages of Philip K. Dick’s Minority Report into the real world. Similarly, China’s “Integrated Joint Operations Platform” combines data from multiple sources, including online tracking and facial recognition-enabled CCTV cameras, as well as health, legal and banking records, in order to flag suspected political dissidents. And all this is planning, too. | |||
The time has come for concrete, rather than abstract, proposals for the democratization of global governance, economics and planning, including around issues of geolocation, social networking, search, data mining, machine learning and ubiquitous computing. Because here’s the thing: the big data cat is out of the bag. Both the ubiquitous surveillance of corporations and the ubiquitous surveillance of the state are already here. We need a third option—one that goes beyond the state-versus-market dichotomy. | |||
== INDEX FUNDS AS SLEEPER AGENTS OF PLANNING == |
Revision as of 20:30, 12 June 2024
THE PEOPLE’S REPUBLIC OF WALMART | |
---|---|
Author | Original by Leigh Phillips and Michal Rozworski. Revised Edition by Comrade Milly Graha |
Written in | 5, March, 2019. Revised 3, August, 2023 |
Publisher | Verso Books. Revised by USU |
Type | Book |
Source | https://clarion.unity-struggle-unity.org/peoples-republic-of-walmart-a-salvageable-trainwreck/ |
Foreword by ProleWiki
Below is an abridged version of "The People's Republic of Walmart" referred to as "The Abridged People's Republic of Walmart". Prolewiki will add original the book in time but have added the Abridged version so that people can disern the essential points of the book without needing to be bogged down in the Liberalism of the original authors, of whom, Leigh Phillips is a Zionist.
Included here also is a critique of the original book by Rachel Nagant.
https://clarion.unity-struggle-unity.org/peoples-republic-of-walmart-a-salvageable-trainwreck/
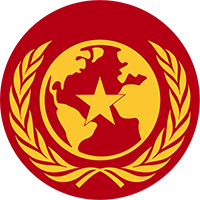
AN INTRODUCTION to ECONOMIC PLANNING, HOLD THE LIBERALISM.
By Leigh Phillips and Michal Rozworski
Revised by Comrade Milly Graham
Note From the Editor
The People’s Republic of Walmart, as originally published by Jakkkobin and Verso Books, was a text rife with internal contradiction. The information about the use of planning in capitalist firms — planning as a subordinate social form within a market economy — is well researched, highly enlightening, and, I believe, imminently important for contemporary revolutionaries to understand. At the same time, however, I could not in good conscience recommend the book to anyone as it was, because its social and political commentary was — pardon my language — quite shit. Kautskyism, Bernsteinism, Lassalleanism, Narodnikism, Proudhonism — the book had it all like a bingo card of revisionism. Writing an entirely new book would be a waste of effort (and a missed opportunity to annoy the authors), so, instead, I have liberated what I believe to be a worthwhile read, particularly for developing Marxists or for a Marxist study group. I won’t claim to have perfectly picked clean every morsel off the bones of the original text, but I think you’ll find this revised edition much more valuable nonetheless. For the most part, edits of the remaining text are stylistic rather than changes to the content, but for the sake of transparency, any sections I have added or substantively altered will be marked with a red asterisk: *
Hugs and kisses,
- Comrade Milly Graham
WHY PLANNING?
There is certainly overlap between the set of all goods and services that are useful to humanity, on the one hand, and the set of all goods and services that are profitable, on the other. You likely find underwear to be a useful product (though for commandos, this is no certainty); The Gap, meanwhile, finds it profitable to produce such a product—a happy coincidence, of which there are many. But the set of all useful things and the set of all profitable things are not in perfect correspondence. If something is profitable, even if it is not useful or is even harmful, someone will continue producing it so long as the market is left to its own devices.
Fossil fuels are a contemporary example of this irremediable, critical flaw. Wonderful though they have been due to their energy density and portability, we now know that the greenhouse gasses emitted by fossil fuel combustion will rapidly shift the planet away from an average temperature that has remained optimal for human flourishing since the last ice age. Yet, so long as governments do not intervene to curtail the use of fossil fuels and build out the clean electricity infrastructure needed to replace them, the market will continue to produce them. Likewise, it was not the market that ended production of the chlorofluorocarbons that were destroying the ozone layer; instead it was regulatory intervention—planning of a sort—that forced us to use other chemicals for our fridges and cans of hairspray, allowing that part of the stratosphere that is home to high concentrations of ultraviolet ray—deflecting tripartite oxygen molecules to largely mend itself. We could recount similar tales about how the problems of urban air pollution in most Western cities or of acid rain over the Great Lakes were solved, or how car accident mortality rates or airline crashes have declined: through active state intervention in the market to curb or transform the production of harmful—but profitable—goods and services. The impressive health and safety standards of most modern mining operations in Western countries were achieved not as a result of any noblesse oblige on the part of the owners of the companies, but rather begrudgingly, as a concession following their defeat by militant trade unions.
Conversely, if something is useful but unprofitable, it will not be produced. In the United States, for instance, there is no universal public healthcare system, though healthcare for all would certainly be wonderfully useful. But because it is not profitable, it is not produced. High-speed internet in rural areas is also not profitable, so private telecommunications companies are loath to provide it there, preferring instead to cherry-pick profitable population-dense neighborhoods. And amid a growing global crisis of antimicrobial resistance, in which microbial evolution is defeating antibiotic after antibiotic and patients are increasingly dying from routine infections, pharmaceutical companies have all but given up research into new families of the life-saving drugs, simply because they are not profitable enough.
That amputation or surgery to scrape out infected areas might return as common medical responses is not a pleasant thought. But this course of action was the only one left to the doctors of nineteen-year-old David Ricci of Seattle when they surgically removed part of his leg, following repeated infections from drug-resistant bacteria—acquired in a train accident in India—that could not be treated, even with highly toxic last resort antibiotics. Each time the infection returned, more and more of the leg had to be cut off. Although Ricci has since recovered, he has lived in perpetual fear of the reappearance of the bugs that can’t be fought. As a 2008 “call to arms” paper from the Infectious Diseases Society of America (IDSA) put it, “[Antibiotics] are less desirable to drug companies and venture capitalists because they are more successful than other drugs.” Antibiotics are successful if they kill off an infection, at which point—days or weeks, or at most months, later—the patient stops taking the drug. For chronic diseases, however, patients may have to take their medicine every day, sometimes for the rest of their lives. Thus, the paper concluded, it is long-term therapy—not cures—that drives interest in drug development. Policy proposals from the likes of the IDSA, the World Health Organization and the European Union amount to begging and bribing the pharmaceutical companies to lift a finger; but even here, however unambitious the approach, it is still external to the market. (Nationalization of the pharmaceutical industry would be cheaper, and a much more rapid and effective approach, but most pundits deem it too “radical,” giving off too much of a whiff of socialism).
Beyond this one sector, we might note that basic blue-sky research in any field simply cannot be done by the private sector, because it is extremely expensive but makes no guarantee of any return on investment. Thus research is almost entirely a phenomenon characteristic of public institutions (or at least public funding). Similarly, it was not the market that got us to the moon, but rather a little ol’ public sector enterprise called NASA. Maybe you’ve heard of it?
*Besides determining what kinds of things are produced, there is another irresolvable, fundamental problem with the market: the propensity towards crisis. The “anarchy of production,” that is, the market system, compels every capitalist, as a compulsory law, to improve his means of production, to drive down the price of his commodities with the development of labor-saving technologies. But the expansion of production inevitably grows faster than the market which must absorb its products for the capitalist to realize his investment. Try as the capitalist might to expand his markets, to expand the sphere of circulation or to drive up consumption with advertising, he will forever be stuck in a tragic “boom and bust” cycle. The forces of production created by society thus overwhelm the form of exchange of society, creating a crisis, not of scarcity, but, absurdly, of overproduction. For the biggest of capitalists, these crises are merely another opportunity to improve their accumulated horde of wealth, to buy out the failing businesses. For the rest of us in society, crisis means losing one's business, one’s job, one’s home, and all the other elements of one’s means of subsistence. It means the destruction of the means of production, or the surplus commodities, resetting the cycle back to the start. This contradiction between the forces of production and the relations of production — the systems of exchange and ownership — cannot be resolved without social revolution, without abolishing the market!
In general, criticisms of the current way of doing things propose that the market be replaced, or at least reined in. But if allocation does not proceed via the market, then it will occur via economic planning, also known as “direct allocation”—made not by the “invisible hand” but by very visible humans. Indeed, this form of planned allocation already takes place widely in our current system, on the part of elected and unelected individuals alike, by both states and private enterprises, and in centralized and decentralized forms. Even arch-capitalist America is home not only to Walmart and Amazon, but also to the Pentagon: in spite of being incredibly destructive, the US Department of Defense is the single-largest employer in the world, and a centrally planned public sector operation. In fact, almost all countries are, to varying degrees, “mixed” economies, making use of both markets and planning.
Indeed, planning has accompanied human societies as long as they have existed. Thousands of years ago, the civilizations of ancient Mesopotamia created a nexus of economic institutions that connected the workshops and temples of the cities to peasant agricultural production in the countryside. The Third Dynasty of Ur (Ur III), which flourished around the Tigris and Euphrates Rivers near the end of the third millennium BCE, was among the first to make the breakthrough to widespread permanent record keeping. Clay tablets from Ur III include predictions of crop yields based on averages of soil quality, themselves derived from years of record keeping. Even though the economy was still at the mercy of uncontrollable weather, it could be managed at a rudimentary level. With the advent of detailed accounts, expectations and approximations—both crucial to planning— became features of economic life. Unlike the localized gift-exchange economy of prehistory, ancient Mesopotamia saw systems of centralized redistribution that mimic today’s welfare states: taxes and levies in, transfers of goods and services out.
Increasingly complex economic record-keeping, accounting and social institutions all point to early ancient civilizations producing something that cannot but be described as economic calculation and planning. This is not to say there was some Arcadia of central planning at this time, any more than it is accurate to describe hunter-gatherer society as some peaceful egalitarian Eden. The planning of the ancients was not only rudimentary and partial; it was also far from being a rational way of securing the shared benefit of all. Indeed, ancient planning was at the service of an economic system created for the benefit of a small coterie of elites who were motivated to maintain their wealth and power. Sound familiar?
There is not only a crying need for us to talk about what an alternative to the market would be, but also a great deal of confusion about what planning is and its history. To take one example: China appears to be the last man standing in the global economy; its growth rates, even if they have declined recently from eye popping to merely gobsmacking, have been achieved through an admixture of free market mechanisms and very heavy shepherding by central planners and party-state managers. It seems even some members of the ascendant bourgeoisie in that country believe that Mao’s economic planning was less mistaken than premature. A 2018 Financial Times feature describes Jack Ma, founder of the Chinese e-commerce colossus Alibaba Group, as part of a growing movement in the People’s Republic who argue that “the fatal flaw of state planning was simply that planners did not have enough information to make good decisions.” He and his co-thinkers believe that “big data” can solve this problem. Could he be right?
In such volatile times, it cannot be ruled out that a socialist revolution might, within our lifetimes, burst forth even within the capitalist heartlands, within the belly of world imperialism. If we do not take pains to sketch out ahead of time what an alternative to the market might look like, those involved will inevitably fall back on versions of what they already know. The capitalist-realist earworm, like the Ceti eel in Star Trek II: The Wrath of Khan, remains wrapped around our cerebral cortex, foreclosing the possibility of transformation even at the moment of its realization.
The time, then, is as ripe as browning avocados on toast to uncover a very old conversation: a long-standing but largely forgotten argument over the question of planning. Our aim is not to offer a comprehensive, definitive survey of this almost century-long discussion, which economists refer to as the “economic calculation debate” (or “socialist calculation debate”)—whether it is mathematically and physically possible to plan an economy, and whether this is desirable—but to provide a plain-language, hopefully even enjoyable, introduction for the uninitiated. In the main, we aim here to bring together and make more easily comprehensible ideas and findings that have been forgotten or are otherwise jargon filled, mathematical, or computer science-oriented, or which lie buried in the pages of little-read operations research or business-management journals. Thus, we lean heavily on the work of economic historians, computer scientists and scholars of commerce. In writing a primer on planning, and on the challenge of logistics and economic calculation, we hope to take this vital debate down from moldering academy shelves and reintroduce it into the field of live political combat.
Above all, our goal with this brief text is simply to flag a rarely recognized, yet obvious, fact that in some sense makes the “calculation debate” anachronistic: it is already the case that great swaths of the global economy are planned. Walmart is a prime example. Thus the question as to whether planning can exist at large scales without crippling economic inefficiencies could be moot. There might be no single machine that we can simply take over, run them with new operators but otherwise leave them unchanged; but there is a foundation of planning that a more just society could surely take up and make its own.
This is not so much a book about a future society, but one about our own. We plan. And it works.
COULD WALMART BE A SECRET SOCIALIST PLOT?
Could Walmart be a secret socialist plot? This is, in effect, the question that Fredric Jameson, American literary critic, Marxist political theorist, and cheeky devil, all too briefly poses in a footnote to his 2005 volume Archaeologies of the Future, a discussion of the nature of utopia in the age of globalization. Mr Jameson, gleefully poking at the progressive consensus that regards Walmart as a globe-barnacling chain of retail hypermarkets, the Galactus of capitalism, the beau idéal—perhaps more so even than Goldman Sachs—of everything that is wrong with everything that is wrong, Jameson wonders whether we might in fact be missing a trick about this transcontinental marvel of planning and logistics:
The literary utopists have scarcely kept pace with the businessmen in the process of imagination and construction…ignoring a global infrastructural deployment in which, from this quite different perspective, the Walmart celebrated by Friedman becomes the very anticipatory prototype of some new form of socialism for which the reproach of centralization now proves historically misplaced and irrelevant. It is in any case certainly a revolutionary reorganization of capitalist production, and some acknowledgment such as “Waltonism” or “Walmartification” would be a more appropriate name for this new stage.
But beyond these comments, the provocation is not fully developed. He lets the suggestion just hang there until the publication five years later of an essay on the subject: “Walmart as Utopia.” Here, he insists more full-throatedly that Walmart is not merely a useful institution from which, “after the revolution,” progressives could (per Lenin) “lop off what capitalistically mutilates this excellent apparatus.” It is not residual of the old society, he says, but rather something truly emergent of the new one yet to be born. Walmart is “the shape of a Utopian future looming through the mist, which we must seize as an opportunity to exercise the Utopian imagination more fully, rather than an occasion for moralizing judgments or regressive nostalgia.” *Jameson could be right in all but one way: if the seeds of a future social form are truly growing within the soil of the biggest capitalist firms, then this is no “utopia,” no arbitrary pipedream, but a very concrete sneak peak at the “real movement” of history.
Jameson compares the emergence of this novel entity to the discovery of a new species of organism, or of a new strain of virus. He delights at the apparent contradiction of how the largest company in the world, even in its full-spectrum dominance—indeed precisely because of this omnipotence—is described by admiring, horrified business writers as a boa constrictor slowly but inexorably strangling market capitalism. But even here, Jameson is still mostly interested in using Walmart as a thought experiment—a demonstration of “the dialectical character of the new reality,” and an example of the notion within dialectics of the unity of opposites: the firm as “the purest expression of that dynamic of capitalism which devours itself, which abolishes the market by means of the market itself.”
Such philosophical flourishes are more than worthwhile, but we are curious about something perhaps a measure more concrete. We want to take Jameson’s provocation beyond a footnote or a thought experiment and, in the light of what we know about Walmart’s operations, revisit a nearly century-old argument between those who favored socialism and those who asserted that capitalism offered the best of all possible worlds. For beneath the threadbare cliché of the maxim that socialism is “fine in theory, but impossible in practice,” there in fact lie claims about economic planning, and about how to calculate an egalitarian distribution of goods and services without need for markets.
Furthermore, the appearance that these claims have been settled by the defeat of the Communist bloc is merely superficial. And counterintuitive as it may seem at first, the infamously undemocratic Walmart, and a handful of other examples we will consider, offer powerful encouragement to the socialist hypothesis that a planned economy—democratically coordinated by ordinary working people, no less—is not merely feasible, but more efficient than the market.
But before we begin to explain how Walmart is the answer, we first have to ask: What is the question?
The Socialist Calculation Debate
Since the neoliberal revolution of the 1970s and its acceleration following the end of the Cold War, economic planning at scale has been widely derided from right to center-left, and planned endeavors such as public healthcare have been under attack from marketization in most countries. In most jurisdictions, the electricity systems that were once in public hands have long since been privatized; therefore governments committed to efforts to decarbonize electricity companies have had little choice but to employ market mechanisms such as emissions trading or carbon taxation, rather than reducing greenhouse gas emissions via democratic fiat—that is, simply ordering the electricity provider to switch to non-emitting fuel sources. Almost everywhere, transportation, communication, education, prisons, policing and even emergency services are being spun off wholly or in part from the public sector and provided instead by market actors. Only the armed forces remain a state monopoly, and here only up to a point, given the rise of private security multinationals such as the notorious G4S and Blackwater (rebranded as Academi since 2011). The handful of social democratic and liberal parties that still defend public healthcare and public education do so while making vague assertions that “government has a role to play” or that “government can be a force for good,” but they don’t really say why. Social democrats today will argue for a mixed economy, or for a mixture of state planning and the market—but again, they do so without saying why. If planning is superior, then why not plan everything? But if some goods and services are better produced by the market than by planning, then what are the attributes of these particular goods and services that make them so? All this activity and argument empty of actual argument reflects a set of policies enacting surrender to an unchangeable status quo, the architects of which only retroactively attempt to transform such capitulation into a coherent ideology. For much of social democracy in the twenty-first century, beliefs follow from policies, rather than policies from beliefs. And while those centrists and conservatives who cheerlead the market stop short of advocating a world where everything is allocated via markets, they still do not offer arguments explaining why their preferred admixture of market and planning is superior. When challenged, they simply describe the current state of affairs: “No economy is completely planned or completely market-based.”
Well, plainly this is true. But again, this gives no explanation as to why their favored configuration is optimal.
Perhaps this is understandable. It seems, at first glance, almost manifest that the market won the Cold War and that planning lost. Yet if the market is conclusively, unassailably, incontestably the optimal mechanism for the allocation of goods and services, then why have the economies of Western nations continued to experience mismatches between what is produced and what is required—mismatches that have led to severe recessions and near-catastrophic economic crises since 1991? Why was the global economy barely (and likely temporarily) saved from a Depression-scale collapse in investment in 2008, not by market mechanisms, but as a result of (modest) Keynesian pump priming? What is the source of economic stagnation since the Great Recession? Why, after three decades of steady decreases in inequality in the West in the post-war period leading up to the 1970s, has inequality in the developed countries grown over the last forty years, triggering an explosion in popular anger, along with hard-right reaction, in country after country? And why can’t the market, left to its own devices, meet the civilizationally existential challenge of climate change? So the question of market versus planning should appear as unresolved as ever.
In the early decades of the last century, the question of whether the market or planning is the optimal mechanism for the allocation of goods and services was widely accepted as unanswered. In the 1920s and 1930s, left-wing economists influenced by Marxism, on the one hand, and rightwing economists of the neoclassical Austrian School, on the other, were engaged in a vigorous discussion—subsequently known as the “economic calculation problem”—over whether economic planning at scale was feasible. At the time, neoclassicals were not arguing from a position of ideological hegemony. The Soviet Union had recently been established, and the war efforts of both the Allies and the Central Powers were expansive exercises in central planning. By the 1930s, the Bolsheviks had rapidly launched a feudal Russia into electrified, industrial modernity, meaning economists who would criticize planning would have to counter what appeared to be substantial evidence in its favor. As a result, partisans on both sides took the idea of planning seriously, and the Austrians had to work hard to try to prove their point, to show how economic planning was an impossibility.
Ludwig von Mises, Austrian School economist and hero of latter-day neoliberals, launched the first counter-volley against the advocates of planning. In his seminal 1920 essay “Economic Calculation in the Socialist Commonwealth,” Mises posed the following questions: In any economy larger than the primitive family level, how could socialist planning boards know which products to produce, how much of each should be produced at each stage, and which raw materials should be used and how much of them? Where should production be located, and which production process was most efficient? How would they gather and calculate this vast array of information, and how could it then be retransmitted back to all actors in the economy? The answer, he said, is that the mammoth scale of information needed—for producers, consumers and every actor in between, and for every stage and location of production of the multitude of products needed in society—is beyond the capacity of such planning boards. No human process could possibly gather all the necessary data, assess it in real time, and produce plans that accurately describe supply and demand across all sectors. Therefore, any economy the size of an entire country that tried to replace the myriad decisions from the multitude of sovereign consumers with the plans of bureaucrats working from incorrigibly flawed data would regularly produce vast, chasm-like mismatches between what is demanded and what is supplied.
These inefficiencies would result in such social and economic barbarities—shortages, starvation, frustration and chaos—that even if one accepts the inevitability of inequalities and attendant myriad other horrors of capitalism, the market will still appear benign by comparison.
Meanwhile, Mises argued that the extraordinarily simple mechanism of prices in the market, reflecting the supply and demand of resources, already contains all this information. Every aspect of production—from the cost of all inputs at all times, to the locations of inputs and products, and the changing demands and taste of purchasers—is implicitly captured by price.
There is much more to the calculation debate, and we’ll briefly outline some of the additional mathematical and computational aspects later on, but for now this theoretical standoff should suffice. It is enough to know that as a result of this impasse, depending on our political persuasions, we have opted either for the information imperfections of the market, or for the information imperfections of planning, without ever resolving the debate. The stalemate could even be tweeted in less than 140 characters: “What about data imperfections leading to shortages?” “Oh yeah? Well what about data imperfections leading to injustices?”
Thus we are stuck. Or so it has seemed for a long time.
Planning in Practice
Walmart is perhaps the best evidence we have that while planning appears not to work in Mises’s theory, it certainly does in practice. And then some. Founder Sam Walton opened his first store, Wal-Mart Discount City, on July 2, 1962, in the non-city of Rogers, Arkansas, population 5,700. From that clichédly humble, East Bumphuck beginning, Walmart has gone on to become the largest company in the world, enjoying eye-watering, People’s Republic of China–sized cumulative average growth rates of 8 percent during its five and a half decades. Today, it employs more workers than any other private firm; if we include state enterprises in our ranking, it is the world’s third-largest employer after the US Department of Defense and the People’s Liberation Army. If it were a country—let’s call it the People’s Republic of Walmart—its economy would be roughly the size of a Sweden or a Switzerland. Using the 2015 World Bank country-by-country comparison of purchasing-power parity GDP, we could place it as the 38th largest economy in the world.
Yet while the company operates within the market, internally, as in any other firm, everything is planned. There is no internal market. The different departments, stores, trucks and suppliers do not compete against each other in a market; everything is coordinated. Walmart is not merely a planned economy, but a planned economy on the scale of the USSR smack in the middle of the Cold War. (In 1970, Soviet GDP clocked in at about $800 billion in today’s money, then the second-largest economy in the world; Walmart’s 2017 revenue was $485 billion.)
As we will see, Walmart’s suppliers cannot really be considered external entities, so the full extent of its planned economy is larger still. According to Supply Chain Digest, Walmart stocks products from more than seventy nations, operating some 11,000 stores in twenty-seven countries. TradeGecko, an inventory-management software firm, describes the Walmart system as “one of history’s greatest logistical and operational triumphs.” They’re not wrong. As a planned economy, it’s beating the Soviet Union at its height before stagnation set in.
Yet if Mises and friends were right, then Walmart should not exist. The firm should long since have hit their wall of too many calculations to make. Moreover, Walmart is not unique; there are hundreds of multinational companies whose size is on the same order of magnitude as Sam Walton’s behemoth, and they too are all, at least internally, planned economies.
In 1970, Walmart opened its first distribution center, and five years later, the company leased an IBM 370/135 computer system to coordinate stock control, making it one of the first retailers to electronically link up store and warehouse inventories. It may seem strange now, but prior to this time, stores were largely stocked directly by vendors and wholesalers, rather than using distributors. Large retailers sell thousands of products from thousands of vendors. But direct stockage—sending each product directly to each store—was profoundly inefficient, leading to regular over- or understocks. Even smaller retailers, who cannot afford their own distribution centers, today find it more efficient to outsource distribution center functions to a logistics firm that provides this service for multiple companies.
Logistical outsourcing happens because it would be far too expensive in terms of labor costs for one tiny store to be able to maintain a commercial relationship with thousands of record labels, and vice versa; but that store can have a relationship with, say, five distributors, each of whom have a relationship with, say, a hundred labels. The use of distributors also minimizes inventory costs while maximizing the variety that a store can offer, at the same time offering everyone along the supply chain a more accurate knowledge of demand. So while your local shop may not carry albums from Hello Kitty Pencil Case Records, via the magic of distributors, your tiny local shop will have a relationship with more record labels than they otherwise could.
In 1988, Procter & Gamble, the detergents and toiletries giant, introduced the stocking technique of continuous replenishment, partnering first with Schnuck Markets, a chain of St. Louis grocery stores. Their next step was to find a large firm to adopt the idea, and they initially shopped it to Kmart, which was not convinced. Walmart, however, embraced the concept, and thus it was that the company’s path to global domination truly began.
“Continuous replenishment” is a bit of a misnomer, as the system actually provides merely very frequent restocking (from the supplier to the distributor and thence the retailer), in which the decision on the amount and the timing of replenishment lies with the supplier, not the retailer. The technique, a type of vendor-managed inventory, works to minimize what businesses call the “bullwhip effect.” First identified in 1961, the bullwhip effect describes the phenomenon of increasingly wild swings in mismatched inventories against product demand the further one moves along the supply chain toward the producer, ultimately extending to the company’s extraction of raw materials. Therein, any slight change in customer demand reveals a discord between what the store has and what the customers want, meaning there is either too much stock or too little.
To illustrate the bullwhip effect, let’s consider the “too-little” case. The store readjusts its orders from the distributor to meet the increase in customer demand. But by this time, the distributor has already bought a certain amount of supply from the wholesaler, and so it has to readjust its own orders from the wholesaler—and so on, through to the manufacturer and the producer of the raw materials. Because customer demand is often fickle and its prediction involves some inaccuracy, businesses will carry an inventory buffer called “safety stock.” Moving up the chain, each node will observe greater fluctuations, and thus greater requirements for safety stock. One analysis performed in the 1990s assessed the scale of the problem to be considerable: a fluctuation at the customer end of just 5 percent (up or down) will be interpreted by other supply chain participants as a shift in demand of up to 40 percent.
Just like the wave that travels along an actual bullwhip following a small flick of the wrist, a small change in behavior at one end results in massive swings at the other. Data in the system loses its fidelity to realworld demand, and the further you move away from the consumer, the more unpredictable demand appears to be. This unpredictability in either direction is a major contributing factor to economic crisis as companies struggle (or fail) to cope with situations of overproduction, having produced much more than they predicted would be demanded and being unable to sell what they have produced above its cost. Insufficient stock can be just as disruptive as overstock, leading to panic buying, reduced trustworthiness by customers, contractual penalties, increased costs resulting from training and layoffs (due to unnecessary hiring and firing), and ultimately loss of contracts, which can sink a company. While there is of course a great deal more to economic crisis than the bullwhip effect, the inefficiencies and failures produced by the bullwhip effect can be key causes, rippling throughout the system and producing instability in other sectors. Even with modest cases of the bullwhip effect, preventing such distortions can allow reduced inventory, reduced administration costs, and improved customer service and customer loyalty, ultimately delivering greater profits.
But there’s a catch—a big one for those who defend the market as the optimal mechanism for allocation of resources: the bullwhip effect is, in principle, eliminated if all orders match demand perfectly for any period. And the greater the transparency of information throughout the supply chain, the closer this result comes to being achieved. Thus, planning, and above all trust, openness and cooperation along the supply chain—rather than competition—are fundamental to continuous replacement. This is not the “kumbaya” analysis of two utopian writers; even the most hard-hearted commerce researchers and company directors argue that a prerequisite of successful supply chain management is that all participants in the chain recognize that they all will gain more by cooperating as a trusting, information-sharing whole than they will as competitors.
The seller, for example, is in effect telling the buyer how much he will buy. The retailer has to trust the supplier with restocking decisions. Manufacturers are responsible for managing inventories in Walmart’s warehouses. Walmart and its suppliers have to agree when promotions will happen and by how much, so that increased sales are recognized as an effect of a sale or marketing effort, and not necessarily as a big boost in demand. And all supply chain participants have to implement data-sharing technologies that allow for real-time flow of sales data, distribution center withdrawals and other logistical information so that everyone in the chain can rapidly make adjustments.
*In short, one of the pitfalls of a perfectly competitive market is that information about the rest of the economy is unavailable to each link in a chain of mutually dependent firms. There’s an analogous pitfall in computer science known as “greedy algorithms.” In a greedy algorithm, one assumes that if they always pick the locally optimal choice at each intermediate branch in a tree of possibilities, then one will eventually reach the globally optimal value. Say, for example, I want to get home in the shortest distance possible, but I have several streets I can go down. If I always take the shortest road that I can see in front of me without ever looking ahead to see if I might reach a dead end or a detour, I am likely to actually extend my trip home. On the other hand, if I had knowledge about the relationships and distances between all the roads, I could plan the shortest trip home even if it meant, at particular steps, choosing the longer of two roads. Like a mature adult capable of delaying gratification, I am able to make a short term sacrifice now to yield a bigger reward later. But, by contrast, our acquaintance the market is rather like a child that keeps failing the marshmallow test; it is a “greedy algorithm” in that it might produce locally optimal solutions (say, for a particular firm), but it will never produce the globally optimal solution (the solution that maximizes utility and minimizes costs for all of society). But market actors are not merely naive, without knowledge about the rest of the “map,” they simply have no choice but to pick the greedy method.
We hear a lot about how Walmart crushes suppliers into delivering at a particular price point, as the company is so vast that it is worth it from the supplier’s perspective to have the product stocked by the store. And this is true: Walmart engages in what it calls “strategic sourcing” to identify who can supply the behemoth at the volume and price needed. But once a supplier is in the club, there are significant advantages. (Or perhaps “in the club” is the wrong phrasing; “once a supplier is assimilated by the WalmartBorg” might be better.) One is that the company sets in place long-term, high-volume strategic partnerships with most suppliers. The resulting data transparency and cross–supply chain planning decrease expenditures on merchandising, inventory, logistics, and transportation for all participants in the supply chain, not just for Walmart. While there are indeed financial transactions within the supply chain, resource allocation among Walmart’s vast network of global suppliers, warehouses and retail stores is regularly described by business analysts as more akin to behaving like a single firm.
Flipping all this around, Hau Lee, a Stanford engineering and management science professor, describes how the reverse can happen within a single firm, to deleterious effect. Volvo at one point was stuck with a glut of green cars. So the marketing department came up with an advertising and sales wheeze that was successful in provoking more purchases by consumers and reducing the inventory surplus. But they never told manufacturing, and so seeing the boost in sales, manufacturing thought there had been an increase in demand for green cars and cranked up production of the very thing that sales had been trying to offload.
The same phenomenon occurs in retail as much as it does manufacturing (and manufacturing is merely another link within the retail supply chain anyway), with Toyota being one of the first firms to implement intra- and inter-firm information visibility through its Walmartlike “Kanban” system, although the origin of this strategy dates as far back as the 1940s. While Walmart was pivotal in development of supply chain management, there are few large companies that have not copied its practices via some form of cross–supply chain visibility and planning, extending the planning that happens within a firm very widely throughout the capitalist “marketplace.”
Nevertheless, Walmart may just be the most dedicated follower of this “firmification” of supply chains. In the 1980s, the company began dealing directly with manufacturers to reduce the number of links within, and to more efficiently oversee, the supply chain. In 1995, Walmart further ramped up its cooperative supply chain approach under the moniker Collaborative Planning, Forecasting and Replenishment (CPFR), in which all nodes in the chain collaboratively synchronize their forecasts and activities. As technology has advanced, the company has used CPFR to further enhance supply chain cooperation, from being the first to implement company-wide use of universal product bar codes to its more troubled relationship with radio-frequency ID tagging. Its gargantuan, satellite-connected Retail Link database connects demand forecasts with suppliers and distributes real-time sales data from cash registers all along the supply chain. Analysts describe how stockage and manufacture is “pulled,” almost moment-to-moment, by the consumer, rather than “pushed” by the company onto shelves. All of this hints at how economic planning on a massive scale is being realized in practice with the assistance of technological advance, even as the wrangling of its infinities of data—according to Mises and his co-thinkers in the calculation debate—are supposed to be impossible to overcome.
Sears’s Randian Dystopia
It is no small irony that one of Walmart’s main competitors, the venerable, 120-plus-year-old Sears, Roebuck & Company, destroyed itself by embracing the exact opposite of Walmart’s galloping socialization of production and distribution: by instituting an internal market.
The Sears Holdings Corporation reported losses of some $2 billion in 2016, and some $10.4 billion in total since 2011, the last year that the business turned a profit. In the spring of 2017, it was in the midst of closing another 150 stores, in addition to the 2,125 already shuttered since 2010— more than half its operation—and had publicly acknowledged “substantial doubt” that it would be able to keep any of its doors open for much longer. The stores that remain open, often behind boarded-up windows, have the doleful air of late-Soviet retail desolation: leaking ceilings, inoperative escalators, acres of empty shelves, and aisles shambolically strewn with abandoned cardboard boxes half-filled with merchandise. A solitary brand new size-9 black sneaker lies lonesome and boxless on the ground, its partner neither on a shelf nor in a storeroom. Such employees as remain have taken to hanging bedsheets as screens to hide derelict sections from customers.
The company has certainly suffered in the way that many other brick-and-mortar outlets have in the face of the challenge from discounters such as Walmart and from online retailers like Amazon. But the consensus among the business press and dozens of very bitter former executives is that the overriding cause of Sears’s malaise is the disastrous decision by the company’s chairman and CEO, Edward Lampert, to disaggregate the company’s different divisions into competing units: to create an internal market.
From a capitalist perspective, the move appears to make sense. As business leaders never tire of telling us, the free market is the fount of all wealth in modern society. Competition between private companies is the primary driver of innovation, productivity and growth. Greed is good, per Gordon Gekko’s oft-quoted imperative from Wall Street. So one can be excused for wondering why it is, if the market is indeed as powerfully efficient and productive as they say, that all companies did not long ago adopt the market as an internal model.
Lampert, libertarian and fan of the laissez-faire egotism of Russian American novelist Ayn Rand, had made his way from working in warehouses as a teenager, via a spell with Goldman Sachs, to managing a $15 billion hedge fund by the age of 41. The wunderkind was hailed as the Steve Jobs of the investment world. In 2003, the fund he managed, ESL Investments, took over the bankrupt discount retail chain Kmart (launched the same year as Walmart). A year later, he parlayed this into a $12 billion buyout of a stagnating (but by no means troubled) Sears.
At first, the familiar strategy of merciless, life-destroying post-acquisition cost cutting and layoffs did manage to turn around the fortunes of the merged Kmart-Sears, now operating as Sears Holdings. But Lampert’s big wheeze went well beyond the usual corporate raider tales of asset stripping, consolidation and chopping-block use of operations as a vehicle to generate cash for investments elsewhere. Lampert intended to use Sears as a grand free market experiment to show that the invisible hand would outperform the central planning typical of any firm.
He radically restructured operations, splitting the company into thirty, and later forty, different units that were to compete against each other. Instead of cooperating, as in a normal firm, divisions such as apparel, tools, appliances, human resources, IT and branding were now in essence to operate as autonomous businesses, each with their own president, board of directors, chief marketing officer and statement of profit or loss. An eye-popping 2013 series of interviews by Bloomberg Businessweek investigative journalist Mina Kimes with some forty former executives described Lampert’s Randian calculus: “If the company’s leaders were told to act selfishly, he argued, they would run their divisions in a rational manner, boosting overall performance.”
He also believed that the new structure, called Sears Holdings Organization, Actions, and Responsibilities, or SOAR, would improve the quality of internal data, and in so doing that it would give the company an edge akin to statistician Paul Podesta’s use of unconventional metrics at the Oakland Athletics baseball team (made famous by the book, and later film starring Brad Pitt, Moneyball). Lampert would go on to place Podesta on Sears’s board of directors and hire Steven Levitt, coauthor of the pop neoliberal economics bestseller Freakonomics, as a consultant. Lampert was a laissez-faire true believer. He never seems to have got the memo that the story about the omnipotence of the free market was only ever supposed to be a tale told to frighten young children, and not to be taken seriously by any corporate executive.
And so if the apparel division wanted to use the services of IT or human resources, they had to sign contracts with them, or alternately to use outside contractors if it would improve the financial performance of the unit— regardless of whether it would improve the performance of the company as a whole. Kimes tells the story of how Sears’s widely trusted appliance brand, Kenmore, was divided between the appliance division and the branding division. The former had to pay fees to the latter for any transaction. But selling non-Sears-branded appliances was more profitable to the appliances division, so they began to offer more prominent in-store placement to rivals of Kenmore products, undermining overall profitability. Its in-house tool brand, Craftsman—so ubiquitous an American trademark that it plays a pivotal role in a Neal Stephenson science fiction bestseller, Seveneves, 5,000 years in the future—refused to pay extra royalties to the in-house battery brand DieHard, so they went with an external provider, again indifferent to what this meant for the company’s bottom line as a whole.
Executives would attach screen protectors to their laptops at meetings to prevent their colleagues from finding out what they were up to. Units would scrap over floor and shelf space for their products. Screaming matches between the chief marketing officers of the different divisions were common at meetings intended to agree on the content of the crucial weekly circular advertising specials. They would fight over key positioning, aiming to optimize their own unit’s profits, even at another unit’s expense, sometimes with grimly hilarious results. Kimes describes screwdrivers being advertised next to lingerie, and how the sporting goods division succeeded in getting the Doodle Bug mini-bike for young boys placed on the cover of the Mothers’ Day edition of the circular. As for different divisions swallowing lower profits, or losses, on discounted goods in order to attract customers for other items, forget about it. One executive quoted in the Bloomberg investigation described the situation as “dysfunctionality at the highest level.”
As profits collapsed, the divisions grew increasingly vicious toward each other, scrapping over what cash reserves remained. Squeezing profits still further was the duplication in labor, particularly with an increasingly top-heavy repetition of executive function by the now-competing units, which no longer had an interest in sharing costs for shared operations. With no company-wide interest in maintaining store infrastructure, something instead viewed as an externally imposed cost by each division, Sears’s capital expenditure dwindled to less than 1 percent of revenue, a proportion much lower than that of most other retailers.
Ultimately, the different units decided to simply take care of their own profits, the company as a whole be damned. One former executive, Shaunak Dave, described a culture of “warring tribes,” and an elimination of cooperation and collaboration. One business press wag described Lampert’s regime as “running Sears like the Coliseum.” Kimes, for her part, wrote that if there were any book to which the model conformed, it was less Atlas Shrugged than it was The Hunger Games.
Thus, many who have abandoned ship describe the harebrained free market shenanigans of the man they call “Crazy Eddie” as a failed experiment for one reason above all else: the model kills cooperation.
“Organizations need a holistic strategy,” according to the former head of the DieHard battery unit, Erik Rosenstrauch. Indeed they do. And, after all, what is society if not one big organization? Is this lesson any less true for the global economy than it is for Sears? To take just one example: the continued combustion of coal, oil and gas may be a disaster for our species as a whole, but so long as it remains profitable for some of Eddie’s “divisions,” those responsible for extracting and processing fossil fuels, these will continue to act in a way that serves their particular interests, the rest of the company—or in this case the rest of society—be damned.
In the face of all this evidence, Lampert is, however, unrepentant, proclaiming, “Decentralised systems and structures work better than centralised ones because they produce better information over time.” For him, the battles between divisions within Sears can only be a good thing. According to spokesman Steve Braithwaite, “Clashes for resources are a product of competition and advocacy, things that were sorely lacking before and are lacking in socialist economies.”
He and those who are sticking with the plan seem to believe that the conventional model of the firm via planning amounts to communism. They might not be entirely wrong.
Interestingly, the creation of SOAR was not the first time the company had played around with an internal market. Under an earlier leadership, the company had for a short time experimented along similar lines in the 1990s, but it quickly abandoned the disastrous approach after it produced only infighting and consumer confusion. There are a handful of other companies that also favor some version of an internal market, but in general, according to former vice president of Sears, Gary Schettino, it “isn’t a management strategy that’s employed in a lot of places.” Thus, the most ardent advocates of the free market—the captains of industry—prefer not to employ market-based allocation within their own organizations.
Just why this is so is a paradox that conservative economics has attempted to account for since the 1930s—an explanation that its adherents feel is watertight. But as we shall see in the next chapter, taken to its logical conclusion, their explanation of this phenomenon that lies at the very heart of capitalism once again provides an argument for planning the whole of the economy.
THE ECONOMICS OF INFORMATION
In the Depression year of 1931, Ronald Coase, a twenty-year-old British economics student, arrived in Chicago to pursue an unusual research project. His question was deceptively simple, although the economics he had been taught apparently didn’t have an answer: “Why are there these ‘islands of conscious power’?…If production is regulated by price movements [and] production could be carried on without any organization at all, well might we ask, why is there any organization?” In other words, if the market is the magic bullet to all human interaction, then even the simplest work tasks—from “stock this shelf” to “format this spreadsheet”—could theoretically be governed by prices on markets rather than by managers giving orders. Somewhat naively, Coase asked, why isn’t everything bought and sold on its own little market? Why are there so many times more Walmarts than there are Sears? Why do companies—from mom-and-pop shops to corporate behemoths—even exist?
Coase argued that Markets introduce a whole web of what he called “transaction costs.” Writing a contract, setting up a market or finding the best price all take up resources and time. So long as the cost of doing all this was cheaper in house than on the market (and it was), it was only rational to keep it in house. So the “free” market isn’t really free either! Coase argued that it only makes sense that some decisions would be left to planning—a decision is made, and it is done. Planning is more efficient—though for Coase, only up to a certain point. Having completed his tour of American business and witnessed its inner workings, upon his return to Britain, he compiled his thoughts in a 1932 lecture to University of Dundee students little younger than himself, although it would be another five years before he published his results.
The resulting text, “The Nature of the Firm”, features a quote from economist Dennis Robertson in which he talks of the curiosity of the very existence of companies, unflatteringly describing them as “islands of conscious power in this ocean of unconscious cooperation, like lumps of butter coagulating in a pail of buttermilk.” But where Robertson had merely remarked upon the mystery, Coase explained it: “Those who object to economic planning on the grounds that the problem is solved by price movements can be answered by pointing out that there is planning within our economic system [that] is akin to what is normally called economic planning.”
He was ignored for his insight. To this day, while hats are now tipped to Coase, and even though planning is plainly ubiquitous, taking place at heretofore unimagined scales, most economists talk very little about it. Economics textbooks offer in-depth explanations of consumer goods markets, the labor market, the money market or even the entire economy as one big market, but little to nothing about the planning inside firms. At best, economists will briefly mention planning, and then only to ridicule it. In much of mainstream economics, the firm is just a mathematical equation that consumes inputs and produces outputs. How it does this is rarely asked; its internal workings are insufficiently interesting. Or sufficiently embarrassing.
The vision of an orderly but completely unplanned market economy is nothing but fantasy. Planning exists in the market system and on a truly enormous scale. Today, the volume of transactions carried out within firms is as large as that carried out between them. Managers
have always been very concerned with planning, but it is only by diving deeply into practical management texts that we can learn about its extent under capitalism. Economists have hidden it behind a tangled web of seeming disorder.
Planning The Market
At the same time that Coase was traveling about, asking corporate managers why they didn’t have markets for moving products from shelves at one end of a warehouse to ones at the other, economists elsewhere were still busy arguing whether it was necessary to have markets at all. As noted earlier, Ludwig von Mises argued in 1920 that socialist planning of an entire economy was impossible because complex economies of the kind we now have need both markets and prices. In his view, markets decentralize the vast troves of information that a single planner couldn’t compile and calculate. Prices, however, make it possible to compare vastly different things; without them, he reasoned, how would planners know the relative worth of things as disparate as a car factory and a ballpoint pen and ultimately decide how many of each there should be? The counterargument that best answered these questions, at least for a while, finally came in 1937 from Polish economist Oskar Lange.
Lange believed that the neoclassical economists’ models of the capitalist economy could be commandeered and repurposed for socialist planning. Under capitalism, when H&M makes too many skinny, off-purple corduroy trousers, its stores eventually drive down the price to entice people to buy them. Demand meets supply when the price falls—at least that’s what happens in theory. In reality, the extra pants can end up in landfills, and H&M’s production for next season can end up moving somewhere with lower wages to make ever lower prices possible. Using the equations of Léon Walras, one of the founders of the neoclassical school, Lange wrote a pamphlet in 1937 that imagined a planned economy, which imitated the market without these downsides. Lange’s fictional socialist planners would manipulate “shadow prices” on paper, rather than waiting for real prices to filter down from cash registers to production decisions. Like a UV light at a crime scene, socialist planning would make explicit all the math that only happened in the background in models of capitalism. Lange answered Mises’s challenge—that prices and markets were necessary to any economic rationality—by incorporating them into a model of “market socialism.”
The key was devising how planners would figure out which shadow prices are the right ones—those that ensure the socialist economy is making enough, but not too much, of everything. For this, Lange repurposed another idea from Walras: tatonnement. In French, Walras’s native tongue, the word means “groping toward.” Walras imagined that markets groped toward the right prices until they found the holy grail of economics: general equilibrium, where all markets are in balance and the amount supplied of every single good or service is exactly equal to the amount demanded. Add some more math, and mainstream economists will tell you that they’ve proven that everyone is also as happy as can be, living in the best of all possible worlds.
Lange imagined that these planners would not merely be simulating the market; in theory, they could actually perform this tatonnement better than markets! People under Lange’s “market socialism” would still go to (government-run) stores to buy consumer goods, signaling to planners what they wanted produced. Producers—all also publicly owned—would aim to produce what the planners translated from consumer demands as efficiently as possible, without needing to leave room for profit after covering costs. As the economy produced things and consumers bought them, central planners would run equations, figure out what there was too much of and what there was too little of, and adjust the “shadow prices” until everything was in sync. Even without all the correct information available at once, Lange’s expected his planners to grope toward equilibrium like markets did under capitalism, only better and faster. And it would only be a matter of time before computers came along that were powerful enough to make the process faster still. Lange spent his final years fascinated by computer science and cybernetics. In one of his last papers, he wrote: “The market process with its cumbersome tatonnements appears old-fashioned. Indeed, it may be considered as a computing device of the pre-electronic age.”
By the time the Second World War began, many classical economists begrudgingly admitted that Lange’s arguments worked—at least in theory. If the socialist system of planning Lange and others described was theoretically possible, then the only question that remained was whether it was feasible. Although corporate and military planners, averse to socialism but intrigued by the power of even the simplest mathematical calculation for resource management and control, were beginning to use crude versions of formalized planning tools, it was difficult to imagine when—if ever—the computing power required for planners to solve Lange’s equations in reasonable time on an economy-wide scale would be available. With seemingly dim prospects for viable application, there was no reason to trumpet the fact that the socialists might be right.
Hayek’s Riposte
Such defeatism alarmed another Austrian economist, Friedrich von Hayek, who, following in the footsteps of Mises, was determined to prove Lange wrong. Hayek is better known today as the godfather of neoliberalism, the pro-market ideology that has come to dominate government policy around much of the world, the first incarnation of which is best exemplified by the administrations of Margaret Thatcher in the UK and Ronald Reagan in the United States during the 1980s. Hayek was explicit about wanting ideological regime change. The postwar welfare state truce between capital and labor had barely been installed when Hayek joined a small group of right-wing radicals to found the Mont Pelerin Society in 1944—a free market think tank before its time. It was integral to their task of reshaping ideology that they have at the ready a rebuke to Lange.
For someone who believed so fervently in capitalism, Hayek offered a very honest picture of the system. Maybe it was precisely because he was so ideologically committed to capitalism that he could talk about its shortcomings—all the ways it deviated from the fantasies of the neoclassical economists with their perfect humans, perfect markets and perfect information. Hayek questioned these central assumptions. People are not hyperrational—we have incomplete, imperfect ideas about the world. Markets are never quite in sync: there is always too much or too little of something. Capitalism is dynamic, a process of constant change rather than a state of equilibrium. On this last point, Hayek agreed with Marx. But as we’ll see, it would take a few decades for the mainstream of economics to embrace such notions.
Hayek was right in rejecting mainstream fantasies. In fact, it was Lange who had underestimated the problems he inherited from the economics of his time — he had merely attempted to replace “capitalist” variables in the equations of dominant neoclassical economics with “socialist” ones. In doing so, he took on all the flawed assumptions of the mainstream model.
Without this baggage, Hayek took a different tack from the silent but grudging acceptance of the mainstream. He rejected Lange’s case outright. Hayek argued that markets—incomplete, permanently off tilt, full of fallible humans—do not just aggregate and calculate information. Markets are producers of information and knowledge. Even if Lange’s market socialism allowed planners to calculate better and faster than did free markets, planning would ultimately still be impossible because planners would not have the information created by market interactions to use in their calculations. Buying and selling may not generate technical and scientific knowledge, but it still creates all that knowledge of “time and place” that is instrumental to making efficient production and distribution decisions. Hayek argued that the problem for planners was not in the “how”—the equations to use—but in the “what”—the data that goes into the equations. The copious information planners need is unavailable before markets work their magic. Decentralization creates coordination: only the market can bring together the information that is normally isolated in the heads of different individuals.
Hayek, however, was writing before the advent of “big data,” which is testing the limits of just how much granular information can be collected. It seems that he also wrote in blissful ignorance of Coase, who had shown just how flimsy the veneer of decentralized decision making really is, even under capitalist markets.
Oddly enough, despite challenging the market socialists head on, Hayek’s ideas were initially ignored, perhaps because they were critical not only of left-wing, but also mainstream economic opinion. At a time when even Richard Nixon was pronouncing that “we are all Keynesians now,” how could their maximalist rhetoric be anything but out of step? The debate on the calculation problem continued to unfold in the pages of obscure economic journals. The world, however, had moved on.
But shortly after Nixon’s startling declaration of allegiance, the existing economic orthodoxies on both sides of the Berlin Wall were violently thrown into question. By the 1970s, “really existing socialism” was mired in economic crisis, its cracks beginning to show. The “free world” was troubled, too, experiencing its most severe economic crisis of the postwar period. Political and economic elites saw in the crisis an opening to unwind their postwar compromise with labor, a compact borne not of love, but out of their fear of revolution. It was in this context that the new heterodoxy championed by Hayek became efficacious outside the walls of the academy at last.
We’ve All Been Misinformed
Joseph Stiglitz, another winner of the Swedish National Bank’s Prize in Economic Sciences in Memory of Alfred Nobel, first made his name by furthering the critique of the assumption of human rationality while still making a case for markets. Distinct from the earlier mythology of a perfectly rational Homo economicus—nowhere to be found in reality, but for so long beloved by economists—the economics of information that Stiglitz helped launch started from the seemingly obvious idea that getting our hands on, and using, information is usually costly, and sometimes impossible. An example economists love to use is the market for private health insurance. There is only so much an insurer can do to see if a person buying insurance is relatively healthy. Developing a better and better picture costs more and more. At some point, the costs prevent further information acquisition from making sense. In the same way, hiring a mechanic to take apart and inspect the engine of a used car to find out if it is a “lemon” can cost more than the car itself. Markets can fail: some people will end up overpaying for health insurance, while others will be uninsured. Your local sketchy used car dealership isn’t likely to be the first place you’d think of as a well-functioning market.
Beyond individual markets, Stiglitz and others were asking a bigger question: What if the entire economy was something of a used car dealership? Once enough examples of failing markets accumulate, the entire system’s efficiency and justice can be called into doubt. In short, the economics of information ultimately challenges the argument that capitalism, despite its flaws, is the best of all possible worlds. However, rather than seeing information problems as a reason to explore collective, democratic decision-making alternatives that could bring people and information together, economists went to work making market theory work in spite of humanity’s imperfections. Since the ’70s, the economics of information has generated ever more crafty schemes for incentivizing people or organizations to do things—all, of course, within the bounds of capitalist markets.
Mechanism design is one such scheme. In this obscure corner of economics, economists drum up—elegant, but often mathematically complex—means to compel people or companies to reveal information that they would otherwise keep secret. A new auction format created by economists in the early 1990s to help the US government sell off cell phone frequencies to telecommunications firms is an exemplary case. The auction had rules designed to force companies to reveal how much the rights to frequencies were really worth to them—lying would see them lose the rights to competitors. The design netted the government hundreds of millions of dollars more than expected and is now commonplace around the world.
Mechanism design is a kind of planning, although a very indirect one. Economic decision making of any kind—whether outright planning or a “designed” market—needs to gather the bits and pieces of information spread between people. But information problems don’t preclude other ways of doing things. Rather than creating a complex process that ultimately benefits a few big players, governments today could choose to run a public cell phone utility, which would constitute one more step on the way to greater socialization. As things stand, however, governments make some money on the auction, but give up control over a valuable resource. This also leaves behind a market dominated by a few big players who can charge famously high prices backed by shoddy customer service.
Mechanism design is just one more example showing that the free market also has to be planned. Real-world markets must be consciously made and remade.
Speaking of Making People Do Things…
One of the few economists before Coase to look inside the black box of business was, as it happens, Karl Marx. Marx saw the firm as an instrument for extracting profit off the backs of workers. He alighted upon a simple fact: workers are paid a wage for their time, not for what they produce. *Unlike a machine, living labor-power has a special property: it produces more than it costs to keep it going. That cost, the price of labor-power, is what the worker is paid as a wage; that which they produce belongs to the capitalist; and the difference between the two (plus material costs) is the surplus value. Because, at any given time, the cost of labor-power, one’s wage, is a fixed value, the boss can extract ever more surplus value out of the worker by making them work longer hours or by increasing the intensity of their labor. Hence the longer and harder the proletarian works, the less they work for themself — to produce the value with which they are compensated — and the more they enrich those who hold power over them.
Coase thought that firms planned simply to save costs. For Marx, it is a consequence of the class struggle. This struggle — between the producers and the owners — determines how everything we produce is divided up between us. The manager’s exercise of central planning over his small province of tyranny is therefore not simply a better means to an end, as Coase thought, but a reflection of the essential antagonism between the classes in society. The more the capitalist gets what he wants, the less the worker does; the dictatorial disciplining of labor is not merely more efficient — though it certainly is that — but an essential method of power and control. The GPS device in the UPS driver’s truck, the call center badge that monitors washroom breaks or the white-collar worker’s app that tracks web browsing history are the “sticks” requiring one does as one is told, to keep the workers producing even when they know it does not benefit them to do so.
Yet for mainstream economists, the confrontation between workers and managers only comes up in the context of “shirking.” Shirking, however, is a very rational response for someone who has little or no say over their work, has no deeper sense of collective responsibility, and knows that the profit from what they do ends up in someone else’s pocket. Shirking is not an innate tendency toward laziness, as the capitalists condescendingly claim, but rather a symptom of the worker’s alienation under capitalism.
In response to any mention of durable human cooperation that is not mediated by markets, in particular by the undisguised incentives provided by the labor market—at their most basic, work or starve—defenders of the market system often bring up “the tragedy of the commons.” This phrase, coined by ecologist Garrett Hardin in a 1968 article in the journal Science, refers to a shared resource inevitably depleted through overuse by individuals acting in their self-interest. The prototypical commons employed to illustrate this tragedy is a plot of open, shared pastureland in a village. If farmers only look out for the cows that are theirs, rather than the entire pasture, each will allow their cows to overgraze, and the land shared in common will quickly turn to dust.
Over the course of her long career, Elinor Ostrom, the only woman to win the “not really a Nobel” prize in economics in its fifty-year existence, did much to debunk this crude story. She compiled evidence of groups stewarding common resources and found that in many cases, the commons not only survived but thrived. Rather than being overrun by unthinking self-interest, shared resources were in reality often governed by complex sets of social rules established over time. Ostrom studied actual shared pasture land in Swiss alpine villages and found it had been preserved for common use for over 500 years. Based on this and other case studies, Ostrom went on to identify conditions that helped protect common resources—among them, participation in decision making by users of the resources, the capacity for monitoring usage, meaningful social sanctions and conflict-resolution mechanisms.
Get the Machine before It Gets You
Despite all the enthusiasm about markets and choice, planning remains the modus operandi of business. What has changed is that the advent of the information technology age has permitted the capture of vast stores of information. What do Facebook and Google do? They prod us, gently and with our own collusion, to reveal information about ourselves. Their business model is the economics of information, come to life. For now, they use the accumulated data to sell ad space—who knew the epitome of high technology would be getting the right people to see ads for novelty “I have a Polish husband and I know how to use him” T-shirts?—but the possibilities are much broader.
Uber and other media darlings of the “sharing economy” combine sussing out information with finding new ways to lower transaction costs. Good capitalists that they are, they’re doing it at the expense of workers and democracy (and other capitalists, namely the venture financiers who continue to pump money into a business like Uber even though it has so far failed to turn a profit). Uber’s rapid expansion stems in large part from its army of well-paid lobbyists, who in turn cajole and threaten city governments behind closed doors into cutting regulations around taxi monopolies.
Uber’s drivers, on the other hand, are poorly-paid “contractors.” No longer classified as workers (except in the UK where courts reinstated their rights as workers), they can make below minimum wage and have few labor rights. As with more and more workers in a range of sectors, they are under constant, nigh on panoptical, surveillance via data. Uber uses a five-star driver rating system in which drivers must maintain an average rating of 4.6 stars to keep driving for the company. Uber can “suggest” certain norms for its drivers to follow (how much to smile, what kind of extra services to offer, and so on), but in reality it is the risk of even one bad rating that quickly prods them to fall into line. Yet there is no top-down rule; when businesses can constantly collect and analyze information, strict management happens from the bottom up. Uber’s business model is to use the economics of information to do more than just sell ad space. The company’s ability to make people do things without telling them explicitly is not unique and is but a refinement of capitalism’s ability to make people complicit in their own unfreedom—a refinement made possible by a greater amount of and greater control over information.
On the other hand, rather than the herald of dystopian workplaces everywhere, Uber is also a natural candidate for a worker co-op. All that Uber provides, after all, is an app; the company is nothing but a middleman. A cooperatively owned network of drivers using a similar app could set pay rates and work rules democratically, in the here and now. A drivers’ co-op would be far superior to the venture capital–fueled behemoth we have today, even if this is a form of enterprise that, while introducing more workplace democracy than is normally possible under capitalism in the short run, is still subject to the same profit-seeking imperatives as any firm within capitalism—imperatives that will prompt self-exploitation in order to compete with other enterprises, thus ultimately undermining these very same democratic impulses.
Similarly, social networks could be run as public utilities rather than as private monopolies—remember that we created public electricity or water works after the failures of nineteenth-century robber baron capitalism. One of the big questions of the twenty-first century will be, who owns and controls the data that is quickly becoming a key economic resource? Will it be the fuel for democratic planning, or instead for a new more authoritarian capitalism? These questions require that we recognize the immense challenges posed by data-driven twenty-first-century capitalism: How could we nationalize multinational corporations that span and disregard national borders, and often play jurisdictions off of one another? How would we ensure privacy with so much data under collective, state control?
Privately held data is making possible more efficient production, but at the same time it is enabling closer supervision, and modern corporate planning is only starting to take advantage of all this newly available information. One outcome is illusory freedom for workers. If we constantly produce information both at and outside of work, we don’t need to be supervised so directly—but the boss is still watching, and doing so more closely than has ever before been possible. Data and metrics speak for themselves: managers can see how many parts a worker assembled per minute or how many packages a driver delivered per hour.
Increasing self-management at work—ostensibly without managers, but still closely surveilled—is a symptom of bigger changes. As wages, both in the United States and across much of the global North, have grown at glacial pace or outright stagnated since the 1970s, workers have taken on more personal debt just to keep up. At the same time, governments have cut public benefits, leaving workers more vulnerable when they are laid off or injured. Even Alan Greenspan, the former head of the US Federal Reserve, called today’s workers “traumatized.” Translated, this means that pressures to fall into line now exist outside the explicit top-down hierarchies.
Capitalism is stuck with planning even though it regularly transmogrifies its techniques of planning. Today, capitalist planning exists both in the old, hierarchical sense that Coase studied as well as in new, more roundabout ways that take cues from the economics of information.
Opening the Gates to the Future
There’s an old quip among historians of economics that a PhD-level microeconomics textbook from the 1960s could be mistaken for a textbook at the department of planning at a university in Havana. In the microeconomics textbook, the free market generates the prices that dictate how much of everything is produced and how things are distributed; in the planning textbook, a planner solves the same equations by coming up with the equivalent proportions of production and distribution. Oskar Lange’s version of socialism and the economic orthodoxy of the twentieth century shared the same flawed assumptions. Over time, as outlined in this chapter, many poked holes in these assumptions: Markets are costly, said Coase. Human beings are not infinitely powered, all-knowing calculators, argued Stiglitz. Even Hayek was right: capitalism is dynamic, not static, and rarely in the sort of equilibrium imagined by Lange and conventional economics.
But the economics of information also challenges Hayek’s counterargument to Lange, that the market is the only means we have to produce all the information that planning would require in the first place. For markets sometimes fail to discover the right information, and that which they do reveal can be false. Also, the enormous amount of economic activity that continues to take place outside the market—within the black boxes we call Walmart or Amazon or General Motors—is evidence against Hayek. At the same time, the rise of information technology shows just how much information it is possible to have at our fingertips. Hayek describes prices as “a system of telecommunications”; today, we have telecommunications far more precise and powerful that can communicate information directly without it being mediated by prices. Hayek’s arguments may have worked to disarm some of Lange’s vision for planning, but they shouldn’t stop contemporary socialists from arguing for democratic planning that is also a process of discovery. Planning is not only possible, but is already all around us, albeit in hierarchical and undemocratic forms. What a very different, democratic planning will look like is a question every contemporary revolutionary should take seriously.
MAPPING THE AMAZON
Amazon is on its way to developing psychic powers. Or at least, such was the fantasy that one could be forgiven for believing, based on the hosanna-filled, adrenalized newspaper column inches that appeared in the summer of 2014 when the online bookseller-turned-“everything store” filed a patent application for a new process it called “anticipatory shipping.” Amazon would soon know what you wanted to buy before you knew it yourself. When you placed an order for the latest John Green young adult novel for non–young adults, another jar of artisanally brined lupini beans, or that Instant Pot wonder–pressure cooker that produces pulled pork faster than the speed of light, the package would already be on its way.
As those journalists less prone to the confection of hyperbolic clickbait pointed out at the time, what this patent describes is in truth a very small step from what Amazon already does. It is a minor extension of the kind of data the company already collects and of the colossal, tentacular logistics operation it already runs. Amazon, building its retail market position on the back of the internet revolution, is the largest technology company using the fruits of modern IT to distribute consumer goods. In short, Amazon is a master planner. It is these sorts of logistical and algorithmic innovations that give the lie to the hoary free market argument that even if planning can deliver the big stuff like steel foundries and railways and healthcare, it would stumble at the first hurdle of planning for consumer items. A fortiori, Amazon offers techniques of production and distribution that are just waiting to be seized and repurposed.
What Amazon Plans
Since its late-’90s dot-com beginnings selling only books, Amazon has expanded to potentially fulfill a large part of a household’s everyday consumption. Echoing Walmart’s horizontal integration, the company has even started to incorporate producers of the things it sells into its distribution network by placing its own workers at the factories and warehouses of some of its key suppliers. Under what the company calls its “Vendor Flex” program, the number of Band-Aids that Johnson & Johnson produces, for example, can depend in part on Amazon’s need. It gives the retail behemoth a role in managing production that extends beyond its own corporate borders.
Beyond simply distributing products, Amazon is, like Walmart, “pulling” demand. In fact, in its early days, Amazon headhunted so many top-level managers from Walmart for their logistics savvy that the Waltons sued. The untold billions of gigabytes of customer data that Amazon collects and the algorithm marvels it uses to parse this data give it an incredibly detailed picture of what people want to buy, and when. Meanwhile, integrating operations with producers ensures that products can be ready in sufficient quantities. Here too, given the sheer scale of this economy, we see the fits and starts of a more integrated model of production and distribution planning, however hierarchical and servile toward its bosses it may be. We might describe Jeff Bezos as the bald, moustache-less Stalin of online retail (editor note: no we may not!).
Yet at heart, Amazon remains (for now) a giant distribution network for consumer goods. The internet age has enabled the rise of a new type of retail model for moving goods from producers to consumers, and Amazon took advantage of this opening better than any of its rivals did. Amazon now controls nearly half of total online retail in the United States. So when Amazon plans, it plans big. Some of Amazon’s planning problems are the same as those faced by other major distribution networks; other problems are entirely novel. In essence though, Amazon’s story is another tale of getting the logistics right—in other words, getting things from point A to point B as cheaply as possible. While this task sounds simple enough, it demands plans for everything from warehouse siting and product organization to minimizing the costs of delivering customers’ packages and shortening delivery routes. Wired magazine describes the company as “a vast, networked, intelligent engine for sating consumer desire.”
Add to this the fact that Amazon, as with every internet company, collects improbable amounts of data on its consumers. A conventional brick-and-mortar store doesn’t know which products you look at, how long you spend looking at them, which ones you put in your cart and then put back on the shelf before arriving at the checkout, or even which ones you “wish” you had. But Amazon does. This data tsunami not only involves consumer information, but stretches throughout the supply chain, and the company uses this data to its advantage wherever it can. Its planning problems are no longer the pedestrian optimization challenges faced by any large company before the internet age, but rather the optimization of “big data”—sets of data that are produced at such gargantuan volumes, varieties and velocities that traditional data processing techniques and software are insufficient.
Amazon’s scale—its ambition to be the “everything store”—introduces significant problems for its IT systems. It is one thing to deliver even a thousand products to a hundred or a thousand retail stores, as would a traditional seller. It is another to deliver millions of products to millions of customers.
The warehouse and transport problems mentioned above are a particular class of mathematical challenge known as “optimization problems.” In an optimization problem, we aim to do something in the best way possible, subject to a number of limits on our action, or “constraints.” Given three different possible routes through a city to deliver a package, say, which is fastest given the number of traffic lights and one-way streets? Or more realistically for Amazon, in delivering some daily number of packages, the company is limited by the schedule of delivery flights, the speed of airplanes, the availability of delivery trucks and a host of other constraints, in addition to city traffic. There are also random events, such as bad weather, that can shut down airports—and while these are sporadic, they are also more likely in some places and at certain times than others.
Every day when you commute to work, you are solving a relatively simple optimization problem. But the math behind optimization is very complex for problems with more than even just a handful of constraints. Given enough variables (conditions that can change) to be optimized and enough constraints, even the most powerful supercomputer we can currently construct, armed with the best possible algorithm we can design, would be incapable of solving some of these problems within our lifetime, and some even within the lifetime of the universe (editor's note: there’s a $1 million reward left unclaimed for anyone who can solve the century-old “traveling salesman” problem!). Many of Amazon’s problems fall squarely into such categories.
So while patents for drone delivery get all the media attention, the true wonders at the heart of its operations are actually the esoteric mathematical techniques that help it manage and simplify its optimization problems. To give one example, these key patents help Amazon plan how to best move items between warehouse shelves and customer doorsteps. Part of solving this problem involves “load balancing”: the same way that your computer shifts tasks so as to not crash any single system, Amazon decides where to build its massive warehouses and how to distribute products between them to make sure no part of its system gets overloaded. To be clear, Amazon’s planning methods are not complete solutions to optimization problems that might take the lifetime of the universe to solve, but instead simply best approximations to get around exploding mathematical complexity. Yet Amazon still chooses to plan rather than leave optimization up to price signals from the market.
Again, take the problem of shipping orders at the lowest cost. Even precisely answering the seemingly simple question of finding the lowest cost shipping method for a day’s worth of orders can quickly grow out of hand. There is no single best way to ship one order out of thousands or millions shipped on a given day, because each order’s cost depends on all the others. Will the plane from the UPS “Worldport” hub in Louisville, Kentucky, to Phoenix be full? Did your neighbor down the street order her electric toothbrush with express shipping, or can it be delivered with your book order tomorrow? The complexity ratchets up still further when Amazon considers not only all the possible alternative routes—which it controls—but also adjusts for the possibility of random events such as severe weather and tries to predict the next day’s orders. This “order assignment” optimization problem has hundreds of millions of variables, and no easy solution. The problem is so complex that there are not even approximations that can take every aspect of the problem into account.
But despite such problems, the planning process within Amazon does not fall apart. While Amazon may depend on horrible working conditions, low taxes and poor wages, it nevertheless functions. The planning problems faced by individual corporations under capitalism do have approximate, “good enough” solutions. As this book argues again and again, planning exists on a wide scale within the black box of the corporation—even if it is “good enough” rather than perfect.
That’s the trick: to find the best possible, even if partial, approximations. Amazon’s modelers work to bring intractably complex problems down to size, to build plans that neither stretch into infinite time, nor respond to all the possible random events that could happen at every step, but that simply work.
Structure amid Chaos
Describing Amazon as a big planning machine doesn’t quite match its image as an icon of “new economy” disruption. Even before Silicon Valley became a hub of global capitalism, planning was typically well hidden behind the facade of competition. Today, the facade has only become more ornate: all you see is a website and then a package at your doorstep. Behind the scenes, however, Amazon appears as a chaotic jumble of the most varied items zipping between warehouses, suppliers and end destinations. In truth, Amazon specializes in highly managed chaos. Two of the best examples of this are the “chaotic storage” system Amazon uses in its warehouses and the recommendations system buzzing in the background of its website, telling you which books or garden implements you might be interested in.
Amazon’s recommendations system is the backbone of the company’s rapid success. This system drives those usually helpful (although sometimes comical—“Frequently bought together: baseball bat + black balaclava”) items that pop up in the “Customers who bought this also bought…” section of the website. Recommendations systems solve some of the information problems that have historically been associated with planning. This is a crucial innovation for dreamers of planned economies that also manage to satisfy consumer wants. The chaos of individual tastes and opinions is condensed into something useable. A universe of the most disparate ratings and reviews— always partial and often contradictory—can, if parsed right, provide very useful and lucrative information.
Amazon also uses a system it calls “item-to-item collaborative filtering.” The company made a breakthrough when it devised its recommendations algorithm by managing to avoid common pitfalls plaguing other early recommendation engines. Amazon’s system doesn’t look for similarities between people; not only do such systems slow down significantly once millions are profiled, but they report significant overlaps among people whose tastes are actually very different (e.g., hipsters and boomers who buy the same bestsellers). Nor does Amazon group people into “segments”—something that often ends up oversimplifying recommendations by ignoring the complexity of individual tastes. Finally, Amazon’s recommendations are not based on simple similarities, such as, in the case of books, keywords, authors or genres.
Instead, Amazon’s recommendation algorithm finds links between items based on the activity of people. For example, a bicycle repair manual may consistently be bought alongside a particular bike-friendly set of Allen keys, even though the set isn’t marketed as such. The two things may not be very obviously related, but it is enough that some people buy or browse them together. Combining millions of such interactions between people and things, Amazon’s algorithm creates a virtual map of its catalog that adapts very well to new information, even saving precious computing power when compared to the alternatives—clunkier recommendation systems that try to match similar users or find abstract similarities.
Here is how the researchers at IBM’s labs describe Amazon’s recommendations: “When it takes other users’ behavior into account, collaborative filtering uses group knowledge to form a recommendation based on like users.” Filtering is an example of an IT-based rejoinder to one of the criticisms Hayek leveled against his socialist adversaries in the 1930s calculation debate: that only markets can aggregate and put to use the information dispersed throughout society. The era of big data is proving Hayek wrong. Today’s deliberately planned IT systems are starting to create “group knowledge” (collective intelligence, or shared information that only emerges out of the interactions within or between groups of people) out of our individual needs and desires. And Amazon doesn’t just track market transactions. Beyond what you buy, the company collects data on what you browse, the paths you take between items, how long you stay on the page of each item you browse, what you place in your cart only to remove it later, and more.
Hayek could not have envisioned the vast amounts of data that can today be stored and manipulated outside of market interactions (and, to be fair, even many Marxists have assumed that the myriad capricious variables associated with faddish consumer items in particular forecloses the capacity for their socialization), although he certainly would have admired the capitalists such as Bezos who own the data and use it to pad their obscene fortunes. It is a delicious irony that big data, the producer and discoverer of so much new knowledge, could one day facilitate what Hayek thought only markets are capable of.
Really, it is not such a big step from a good recommendation system to Amazon’s patent for “anticipatory shipping.” It has a viability beyond any Silicon Valley, TED Talk–style huckster bombast or tech-press cheerleading. The reason this genuinely incredible, seemingly psychic distribution phenomenon could actually work is not a result of any psychological trickery, subliminal advertising craftiness, or mentalist power of suggestion, but is found in something much more mundane: demand estimation. With its huge data sets that measure the relationships between products and people, Amazon is already very successful in figuring out demand for particular products, down to a previously unimagined level of detail.
In our irrational system, the ultimate purpose of product recommendations is to drive sales and profits for Amazon. Data scientists have found that rather than high numbers of customer-submitted reviews, which have little impact, it is recommendations that boost Amazon’s sales. Recommendations help sell not only less popular niche items—when it’s hard to dig up information, even just a recommendation can be enough to sway us—and bestsellers that constantly pop up when we’re browsing.
Zooming out beyond Amazon’s corporate interests, the recommendations system is a way of managing and integrating great swaths of social labor. Many of us freely, without expectation of any reward, spend time and energy writing reviews and giving out stars to products or even just mindlessly browsing on Amazon and other technology platforms. This is work that we and others benefit from. Even over the course of one day, we may repeatedly engage in unpaid labor to rate everything from the relatively innocuous, such as call quality on Skype, to the more serious, such as posts, comments and links on Facebook and Twitter, to the potentially very impactful on individual lives, such as the “quality” of Uber drivers. Under capitalism, the social labor of many is transformed into profit for the few: the filtering may be “collaborative,” but the interests it serves are competitive and very private.
Workers Lost in the Amazon
While many of us end up using free time to perform the social labor that allows Amazon to perfect its recommendations system, Amazon’s warehouses run on paid labor that is nonunionized and frequently occurs under appalling, similarly big data–disciplined conditions. Before taking a closer view of the work itself, let’s quickly look at the workplace. The focal points of Amazon’s distribution network are its warehouses, which the company calls “fulfillment centers.” These usually take up football fields’ worth of floor space jammed with shelving units. Amazon uses a peculiar form of organization called “chaotic storage,” in which goods are not actually organized: there is no section for books or subsection for mystery fiction. Everything is jumbled together. You can find a children’s book sharing a bin or shelf with a sex toy, caviar next to dog kibble.
Once again, powerful planning is what allows Amazon to save on what turns out to be needless warehouse organization. Every item that enters a fulfillment center gets a unique barcode. Once inside the warehouse, items go in bins, each of which also has a unique code. Amazon’s software tracks both the items and the bins as they move through the warehouse. The software always knows which bin an item is in and where that bin is. Because items can always be found easily, deliveries from suppliers can be unloaded where it is convenient, rather than methodically organized and reorganized.
Amazon’s chaotic storage could be a metaphor for the free market system: at first glance, it seems that the chaos organizes itself. Orders and packages zoom through the system and customers get what they want. But as with the free market, upon closer inspection we see thickets of deliberative planning at every step. Highly refined IT systems make sense of the chaotic storage, track items from the moment they arrive at a warehouse to the moment they leave, and make sure everything falls seemingly supernaturally into place. Everything ordered, coordinated, planned and not a market in sight to perform any of these billions of allocation decisions.
Planning is also present in the most minute details of a warehouse worker’s day. Handheld scanning devices tell workers where to go to pick items for orders. Workers are appendages of machines that lay out precisely which routes to follow between shelves and how long they should take. Here’s how a BBC undercover worker-reporter described the work: “We are machines, we are robots, we plug our scanner in, we’re holding it, but we might as well be plugging it into ourselves.” A leading UK researcher on workplace stress contacted by the same BBC investigation claimed that conditions at Amazon warehouses pose serious physical and mental health risks.
Around the start of this decade, Amazon’s top operations managers determined that its warehouses were still too inefficient, and so they themselves went shopping for something better. In 2012 Amazon bought Kiva Systems, a robotics firm, and it now uses robots to put its entire shelving system into motion. Amazon’s updated, even more automated fulfillment centers now feature shelves that move and humans who stand in place—the opposite of what a warehouse normally looks like. Flat, Roomba-like robots rove the warehouse floor along designated pathways. They can lift entire shelving units just off the ground and maneuver them along the same pathways to “picking stations.” These are small designated areas where human order pickers stand, taking items from storage bins and putting them into order bins as shelving units come and go. Frederick Taylor would be proud!
The social, physical and mental cost of a machine for delivering the right things to the right people ultimately falls on the workers who make the machine hum—regardless of whether workers are piloted around a maze of shelves by a handheld scanner or pick orders in place while robots whiz to and fro toward them. The boosters at Wired magazine are in awe of the subjugation of the Chaplins in this twenty-first-century Modern Times: “The packing stations are a whirl of activity where algorithms test human endurance.”
Other more critical reporting has been less kind to Amazon in fleshing out just what these endurance tests entail. In 2011 the Lehigh Valley, Pennsylvania, local paper, the Morning Call, investigated its nearby Amazon fulfillment center. Workers said they routinely faced impossible-to-meet targets, debilitating heat and constant threats of being fired. On the hottest days of the year, Amazon had paramedics on hand outside the warehouse to treat heat-exhausted workers—a cheap Band-Aid solution for Amazon that makes clear its low estimation of health and safety; apparently humane working conditions are not one of its algorithms’ optimization constraints. It was only after this story blew up in the national media and the revelation hurt its largely liberal-tech-and-innovation brand image that Amazon began to refurbish some warehouses with air conditioning. In fact, only one out of the twenty workers featured in the Morning Call story said Amazon was a good place to work.
Amazon workers interviewed by the media consistently report feeling the constant stress of surveillance. Being too slow to pick or pack an item, or even taking a bathroom break that is too long, results in demerit points. Amassing enough of these points can lead to being fired. Soon, this feeling of constant surveillance could become far more visceral: in February of 2018, Amazon patented a wristband that, like an episode of Black Mirror, monitors a warehouse worker’s every hand movement in real time. And Amazon pits workers not only against the clock, but also against one another. Utilizing the lumpenized and semi-proletarian masses from among the industrial reserve army, Amazon staffs warehouses with a mix of permanent and temporary workers hired by subcontractors. Permanent positions are few, but they come with some security, slightly higher pay and limited benefits; they are dangled as carrots before temporary workers, encouraging competition and overwork, further fostering a climate of uncertainty and fear.
With the help of robots, the average time to fill an order in a warehouse automated by Kiva technology has plummeted from ninety minutes down to fifteen. Working conditions, however, haven’t budged: the work remains as dull and draining as ever, warehouses remain hot, and the pace of work can be absurdly fast, regardless of the level of automation. While workers in automated warehouses stand all day and try to keep up with the robots zooming by, workers in the non-automated warehouses can expect to walk nearly double the distance on a daily shift of a typical mail carrier. Even small things like distances to break rooms can be an obstacle—sometimes so long that going both ways can take up most of a break.
Long hours for low pay are the norm in an Amazon warehouse, but the relatively highly paid white-collar workers at Amazon also face a crushing work environment. A 2015 New York Times exposé revealed an environment of overwork and “purposeful Darwinism” that pushes many past their physical and emotional limits. Even if sophisticated planning is Amazon’s workhorse, it is implemented within the bounds of a ruling ideology of ruthless competition that breaks white-and blue-collar workers in different ways. Put differently, Amazon is doing exactly what Marx described in a passage from The Communist Manifesto that often goes underappreciated: “The bourgeoisie cannot exist without constantly revolutionizing the instruments of production, and thereby the relations of production, and with them the whole relations of society.” Our task must be to disentangle the good brought by technology from the tentacles of a social system that degrades workers and subverts more rational planning.
Amazonian Technologies beyond Amazon
Despite being a model of the new, disruptive, internet-dependent capitalism, Amazon remains a planning device as much as other companies ever have. In simplest terms, Amazon is a giant planned machine for distributing goods. It is a mechanism for forecasting, managing and meeting demand for an incredibly wide array of things we need and want. It is a collection of thousands of interlocking optimization systems that work together to carry out the deceptively simple task of moving objects from producers to consumers. Rather than the anarchy of the market, once we enter the Amazon, we are entering a sophisticated planning device—one that offers not only clues for how we could manage demand and supply of consumer goods in a society not built on profit, but also warnings to would-be planners for the public good.
Instead of optimizing the satisfaction of our needs and desires, as well as workers’ working conditions and livelihoods, Amazon’s plans are geared toward maximizing profit for its shareholders—or future profit, since Amazon keeps plowing money from sales into research, IT and physical infrastructure to squeeze out competitors. Planning for profit is in fact an example of capitalism’s web of allocation inefficiencies. The planning technologies dreamed up by Amazon’s engineers are a way of meeting a skewed set of social needs—one that ends up enriching a few, misusing substantial free social labor, and degrading workers. A democratized economy for the benefit of all will also need institutions that learn about people’s interests and desires, optimize via IT systems, and plan complex distribution networks; but they will look different, perhaps alien to the systems we have today, and they will strive toward dissimilar goals.
Three challenges should give us pause before even beginning to call the riddle of democratic planning solved.
First, there is large-scale technical feasibility. The difficulty of planning and optimizing even the isolated task of delivering Amazon’s packages demonstrates that designing systems for economy-wide planning will be anything but trivial. The algorithms that power everything from Amazon’s recommendation system to Google’s search engine are still in their infancy—they are relatively simplistic, making best-estimate guesses, and are prone to failure. Algorithms run into systemic problems, for example with working class and poor people who more frequently use shared devices to shop or non-English speakers, where their capacity for “reading” nuance is limited. We’ll have to storm both the barricades and the optimization problems.
Second, the planning done by Amazon and others still relies heavily on prices in interactions that take place beyond the borders of the firm itself. Amazon purchases its inputs—from the multitude of items it stocks, to the warehouse shelves they sit on, to the servers that run its database—on a market; consumers, meanwhile, also take into account the relative costs of items when deciding whether to add them to their virtual carts. Beyond the confines of the firm, a market system continues to operate. This means that it’s not simply a matter of repurposing existing technologies, lopping off the bosses and otherwise keeping everything the same. Even though there is market-less planning within corporations, it is a form of hierarchical, undemocratic planning that is very much necessary to survive and thrive in a market. Many elements of this planning apparatus, their very form and purpose, are conditioned by that undemocratic hierarchy.
Finally, while the big data collected and processed by Amazon is precisely the kind of tool that would aid in overcoming these challenges of large-scale economic calculation—and indeed it is already being used in this way by the Amazons and Walmarts (never mind the Facebooks and Googles) of the world—we have to recognize that alongside the staggering freedom-enhancing potential of the massive data sets held by both corporations and states, there also lies a staggering capacity for freedom restriction.
The story of Walmart’s major rival Target sending deals on diapers and baby food to several expectant mothers who did not yet themselves know they were pregnant, based on data mined on individual spending patterns, seems almost quaint today. Now, we are only a single Google search for “poor sleep” away from months of bombardment by mattress ads on every social media network to which one belongs. There are more insidious examples: in 2012, the short-lived “Girls Around Me” app used a mash-up of geolocation and social media data to allow individuals to find out all kinds of personal details about women in their vicinity who had used Facebook or Foursquare’s “check-in” feature. The UK’s Daily Mail called it the “Let’s Stalk Women” app, while science fiction author Charles Stross imagined a near future of other, far-nastier data mash-ups—could neoNazis create a “Jews Near Me” app? Beyond the private sector, states across the world are also increasingly using and misusing big data. Police departments across the United States have begun to experiment with something called “predictive policing” to devise methods for predicting offenders, victims, identities, and locations of crimes. It is the arrival of “pre-crime” from the pages of Philip K. Dick’s Minority Report into the real world. Similarly, China’s “Integrated Joint Operations Platform” combines data from multiple sources, including online tracking and facial recognition-enabled CCTV cameras, as well as health, legal and banking records, in order to flag suspected political dissidents. And all this is planning, too.
The time has come for concrete, rather than abstract, proposals for the democratization of global governance, economics and planning, including around issues of geolocation, social networking, search, data mining, machine learning and ubiquitous computing. Because here’s the thing: the big data cat is out of the bag. Both the ubiquitous surveillance of corporations and the ubiquitous surveillance of the state are already here. We need a third option—one that goes beyond the state-versus-market dichotomy.